Lighthouse Blog
Read the latest insights from industry experts on the rapidly evolving legal and technology landscapes with topics including strategic and technology-driven approaches to eDiscovery, innovation in artificial intelligence and analytics, modern data challenges, and more.
Thank you! Your submission has been received!
Oops! Something went wrong while submitting the form.
Thank you! Your submission has been received!
Oops! Something went wrong while submitting the form.
Blog
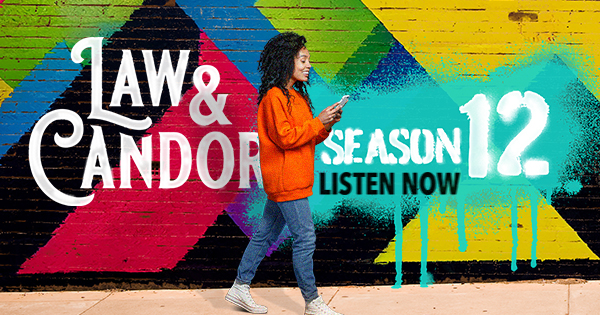
Law & Candor Season 12: Five Views of Innovation and Risk Impacting AI, eDiscovery, and Legal
AI, generative AI, antitrust, second requests, HSR, eDiscovery, review, information governance, healthcare, legal operations, law firm, corporate counsel ai-and-analytics; compliance; corporate; corporate-legal-ops; data-analytics; healthcare; healthcare-litigation; innovative-technology; innovation; information-governance; law-firm; mergers; modern-data; phi; pii; podcast; self-service, spectra; regulation; production mitch montoya In a year of unprecedented advancement in AI capabilities and economic uncertainty, legal teams and attorneys have been given both a compelling look into what the future of their work may look like and a sharp picture of today’s challenges. With a critical eye on how to manage and capitalize on these dueling perspectives that define legal’s current landscape, the guests on the new season of Law & Candor offer insights on a range of issues, including generative AI, new M&A guidelines and HSR rules, collaboration data, strategic partnerships, and the future of the industry. Listen for news, AI and technology updates, and best practices from leaders confronting these challenges and charting new paths forward. Episode 1: The Power of Three: Maximizing Success with Law Firms, Corporate Counsel, and Legal Technology Episode 2: What You Need to Know About the New FTC and DOJ HSR Changes Episode 3: Why Your eDiscovery Program and Technology Need Scalability Episode 4: Generative AI and Healthcare: A New Legal Landscape Episode 5: The Great Link Debate and the Future of Cloud Collaboration To keep up with news and updates on the podcast, follow Lighthouse on LinkedIn and Twitter . And check out previous episodes of Law & Candor at lighthouseglobal.com/law-and-candor-podcast. For questions regarding this podcast and its content, please reach out to us at info@lighthouseglobal.com.
AI and Analytics
Antitrust
Chat and Collaboration Data
eDiscovery and Review
Information Governance
Blog
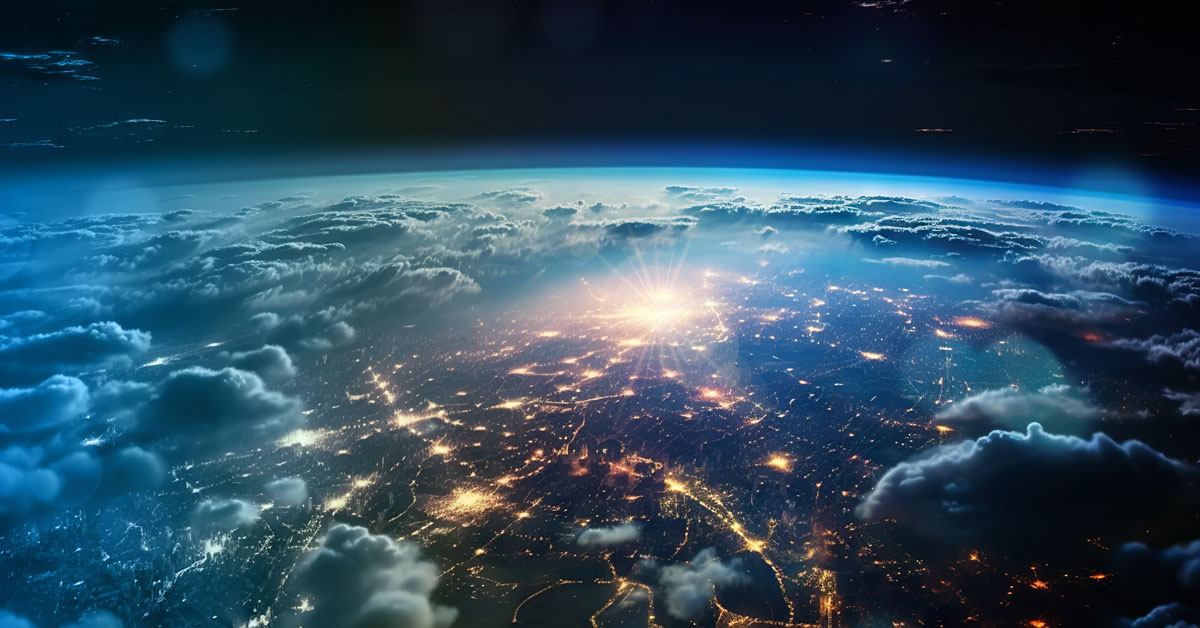
Navigating Cross-Border eDiscovery Issues in the Wake of a U.S. Adequacy Determination
At Lighthouse our teams have the benefit of working across numerous clients, cases, and jurisdictions. As a result, we are building deep institutional knowledge across many aspects of eDiscovery that may be more difficult for individuals or teams to amass organically. To benefit our clients, we regularly share these insights in an ongoing series of best practices articles. This article provides updated guidance on cross-border eDiscovery in the wake of a recent adequacy determination by the European Commission for EU-US data transfers.Best Practices to Support Cross-Border Data Transfers in eDiscovery In any matter that potentially involves the processing and transfer of personal data across country borders, case teams should consider the following factors before deciding on a strategy:The underlying company’s own policy governing the processing of personal data (including transfer mechanisms, such as consent and/or binding corporate rules)The specific countries at issue (some countries have additional requirements for data residency, heightened consent requirements, etc.)The nature of the data (including special categories of protected data, i.e., high risk data), as well as the importance of the custodian and uniqueness/criticality of the dataThe options and feasibility of obtaining custodian consent for the transfer of their data (e.g., time to obtain consent, employment status of the custodian, the impact of obtaining consent on an investigation)When evaluating options for where the data should be processed, case teams should also consider:The country where most custodians are located (i.e., where the largest volume of data will be located) Data center options (if no data center, consider other cloud based or remote kit options and the impact on downstream search/review)The pros and cons of processing data in a single data repositoryMinimization at the point of collection as opposed to once data is processed into a review toolNote that most clients follow a “hub-centric” approach and process data in accordance with specific regions, e.g., data stored in the US is processed in the US; data stored in Europe is processed in a European data center; data stored in APAC is process either in APAC, depending on the country-specific laws, or in Europe, and so forth.Whenever non-U.S. data is present in a matter, case teams should consider the following best practices for cross-border data transfers:Establish lawful grounds for processing personal data (e.g., custodian consent, adequacy decision, or a legal exception defined by applicable data privacy regulations, such as the GDPR’s legitimate business interest exception). Note that many case teams choose not to rely solely on custodial consent for larger matters, unless the data originates from a highly restrictive jurisdiction (e.g., Switzerland, France, Germany, Luxembourg, etc.) or the matter involves specially protected data. Ensure there are adequate safeguards in place to support exceptions, such as the legitimate business interest exception. At a minimum, this includes efforts to “minimize” what is being processed (i.e., collecting only data that is necessary for the activity at hand). Case teams can minimize the volume of data being processed by using keywords or other filters to reduce what is collected, culling data at the processing stage, conducting a search for certain categories of personal data, redacting personal data, and permitting a custodian to review data prior to transfer.Case teams should also follow specific best practices when encountering any of the below scenarios during eDiscovery: Matters involving U.S. litigations and eDiscovery: Consider adding supplemental data privacy safeguards, including putting a protective order in place that specifically addresses the handling of personal data subject to applicable law (e.g., GDPR and other applicable country specific regulations). This includes provisions to designate certain data as subject to the protective order and specific provisions that require the deletion of data (and confirmation of deletion) once the litigation concludes. Matters involving cross-border transfers from other (non-U.S.) countries: Ensure an appropriate cross-border transfer mechanism is in place for all data transfers. Common examples of appropriate cross-border transfer mechanisms include model contract clauses, intra-company agreements, and adequacy decisions rendered by the European Commission (including the adequacy decision for the new EU-U.S. Data Privacy Framework).Matters involving data originating in China (PRC): Take into consideration all data security implications and PRC laws before transferring any data out of the country (including the requirement to conduct a state-secrets review in-country before any data can be transferred outside the country).Matters involving data originating in countries with heightened privacy restrictions and/or sector-specific requirements (i.e., bank secrecy): Consider processing (and potentially reviewing) data in-country.Document the protocol adhered to for each matter.ConclusionWhile transferring personal data across borders may feel like an increasingly complicated task for legal and eDiscovery teams, it is also a task that will be increasingly necessary as corporate data volumes grow and spread. The good news is that case teams do not have to navigate those complexities alone. An experienced eDiscovery partner with a global footprint and information governance/legal experts on staff can work closely with both outside and in-house counsel to develop a solution for cross-border data transfers that meets the legal requirements and needs of each matter. resource-article; data-privacy; information-governanceCross-border data transfercross-border-data-transfersjamie brown
Resource Article
Data Privacy
Information Governance
Blog

Why Legal Teams Need to Reduce Repeated Document Review
Similar matters often pull in the same documents for review during eDiscovery. Many legal teams default to manually reviewing these documents for each matter, but this is quickly becoming untenable.Legal teams can reduce the burden of repeated review through the application of advanced technology and proactive review strategies. They may encounter barriers, from limitations of their current tools to concerns about defensibility. But legal teams can take small steps now that overcome these barriers and prepare them to meet the time, budget, and other pressures they face today.Repeated review exacerbates today’s challengesWe're approaching a time when legal teams simply can’t afford to review the same documents multiple times across matters. The size of modern datasets requires teams to reduce eyes-on review wherever possible. Meanwhile, repeated review of the same documents opens the door to inconsistency, error, and risk.When teams succeed in reducing repeated review, they turn their most common pain points into new sources of value. They get more out of their review spend, help review teams work faster, and achieve the accuracy that they expect and the courts demand.In an earlier post, we dig more deeply into when repeated review happens, what it costs, and how technology can support a different approach. If you’re eager to explore solutions, that’s a great place to start.But many legal professionals are unable to think about solutions yet. They face a range of internal and external barriers that make it hard to move or even see past the status quo of repeated review.If that’s the boat you’re in, keep reading.Changing your approach may appear dauntingLegal teams often have solid reasons for persisting with repeated review. These include:Feasibility concerns Every matter is unique, and teams may assume this means nothing of value carries over from one matter to another.Attorneys may distrust the decisions or data practices associated with prior matters. They prefer starting over from scratch, even if it means repeating work.Technology barriers Legacy tools and software lack the advanced AI necessary to save work product and apply learnings from matter to matter, but adopting new technology takes time and money that legal teams are wary of spending.Companies who use multiple vendors and eDiscovery review teams may store their data in multiple places, making it hard to reuse past work product.It’s true that no two matters are exactly alike, adopting new technology can be challenging, and it’s hard to trust the reliability of something you’ve never used before. But this doesn’t mean that repeated review is still the best option. As shown above, the costs are simply too high.So, what do we do about these barriers? How are teams supposed to move past them? The answer: One step at a time.Explore what’s possible by starting smallThese barriers are most formidable when you imagine rethinking your entire review approach. The idea of looking for potential document overlap across a huge portfolio, or finding and implementing a whole new technology suite, may be too overwhelming to put into action.So don’t think of it that way. Take small steps that explore the potential for reducing repeated review and chip away at the barriers holding you back. Instead of “all or nothing,” think “test and learn.”Look ahead to future matters, perform hybrid QC on past decisionsTo explore the feasibility of reducing repeated review, look at one matter with an eye on overlap. Does it share fundamental topics or custodians with any recent or future matters? Is it likely to have spin-off litigations, such as cases in other jurisdictions or a civil suit that follows a federal one?To build trust in decisions made during prior matters, try performing QC with attorneys and technology working in tandem. This can provide a quick and informative assessment of past decisions and calibrate your parameters for review going forward.Find a technology partner who meets you where you areIf your team lacks the technology to reuse work product, the right partner can right-size a solution for your needs and appetite. The hybrid QC example above applies here too. Many legal teams find that QC is an ideal venue for assessing the performance of advanced AI and getting a taste for how it works, because it’s focused, confined, and accompanied by human reviewers. From there, your team might expand to using advanced AI on a single matter, and eventually, on multiple matters. In all cases, your partner can do the heavy lifting of operating the technology, while explaining each step along the way, with enough detail that you can articulate its use and merits in court (or can “tag in” to present that explanation for you). “The right partner” in this context is someone with the data science expertise to apply the technology in the ways you need, along with the legal experience to speak to your questions and need for defensibility.Likewise, when data or case work are spread across multiple teams and locations, a savvy partner can still find ways to avoid duplicate work. This story about coordinating review across 9 jurisdictions is a great example.Take your time—but do take actionThe beauty of starting small is how it respects both the need to improve and the difficulty of making improvements. Changing something as intricate and important as your document review strategy won’t happen overnight. That’s okay. Take your time. But don’t take repeated review as a given. It threatens timelines, budgets, and quality. And it’s not your only option.For more on the subject, including specific scenarios where teams can reduce repeated review, see our in-depth primer.ediscovery-review; ai-and-analyticsreview; ai-and-analytics; ediscovery; ediscovery-processsarah moran
eDiscovery and Review
AI and Analytics
Blog

3 Reasons Traditional Document Review Isn’t Flexible Enough for Your Needs
Modern data volumes and complexity have ushered in a new era of document review. The traditional approach, in which paid attorneys manually review all or most documents in a corpus, fails to meet the intense needs of legal teams today.Specifically, legal teams need to:• Scale their document review capability to cover massive datasets• Rapidly build case strategy from key information hidden in those datasets• Manage the risk inherent in having sensitive and regulated information dispersed across those datasetsAdvanced review technology—including AI-powered search tools and analytics—enables teams to meet those needs, while simultaneously controlling costs and maintaining the highest standards of quality and defensibility. Rather than ceding decisions to a computer, reviewers are empowered to make faster decisions with fewer impediments. (For a breakdown of how technology sets reviewers up for success, see our recent blog post). In a nutshell, legal teams that use advanced technology can be more flexible, tackling large datasets with fewer resources, and addressing strategy and risk earlier in the process.A flexible approach to scale: refining the responsive setResponsiveness is the center of all matters. With datasets swelling to millions of documents, legal teams must reduce the responsive set defensibly, cost-efficiently, and in a way they can trust.With traditional eyes-on review, the only way to attempt this is to put more people or hours on the job. And this approach requires reviewers to make every coding decision, which is often mentally taxing and prone to error. Advanced review technology is purpose-built to scale for large datasets and provide a more nuanced assessment of responsiveness. Namely, it assigns a probability score—say, a given document is 90% or 45% likely to be responsive—that you can use to guide the review team. Often this means reviewers start with the highest-probability docs and then proceed through the rest, eventually making their way through the whole corpus. But legal teams have a lot of flexibility beyond that. Combining machine learning with rules-based linguistic models can make responsive sets vastly more precise, decreasing both risk and downstream review costs.Using this approach, machine learning is leveraged for what it does best—identifying clearly responsive and clearly non-responsive materials. For documents that fall in the middle of machine learning’s scoring band—those the model is least certain about—linguistic models built by experts target responsive language found in documents reviewed by humans, and then expand out to find documents with similar language markers. This approach allows legal teams to harness the strengths of both computational scalability and human reasoning to drive superior review outcomes.A flexible approach to strategy: finding key documents fasterOnly about 1% to 1.5% of a responsive set consists of key documents that are central to case planning and strategy. The earlier legal teams get their hands on those documents, the sooner they can start on that invaluable work.Whereas it takes months to find key documents with traditional review, advanced technology shortens the process to mere weeks. This is because key document identification utilizes complex search strings that include key language in context. For example, “Find documents with phrase A, in the vicinity of phrases B, C, and D, but not in documents that have attributes E and F,” and so on. A small team of linguistic experts drafts these searches and refines them as they go, based on feedback from counsel. In one recent matter, this approach to key document identification proved 8 times faster than manual review, and more than 90% of the documents it identified had been missed or discarded by the manual review team.The speed and iterative nature of this process are what enable legal teams to be more flexible with case strategy. First, they have more time to choose and change course. Second, they can guide the search team as their strategy evolves, ensuring they end up with exactly the documents they need to make the strongest case.A flexible approach to risk: assessing privilege and PII sooner and more cost effectivelyReviewing for privilege is a notoriously slow and expensive part of eDiscovery. When following a traditional approach, you can’t even start this chore until after the responsive set is established.With advanced technology, you can review for privilege, PII, and other classifications at the same time that the responsive set is being built. This shortens your overall timeframe and gives you more flexibility to prepare for litigation.Legal teams can even be flexible with their privilege review budget. As with responsiveness, advanced technology will rate how likely a document is to be privileged. Legal teams can choose to send extremely high- and low-scoring documents to less-expensive review teams, since those documents have the least ambiguity. Documents that score in the middle have the most ambiguity, so they can be reviewed by premium reviewers.It’s all about options in the endBroadly speaking, the main benefit of supporting document review with advanced technology is that it gives you a choice. Legal teams have the option to start key tasks sooner, calibrate the amount and level of eyes-on review, and strategize how they use their review budgets. With linear review, those options aren’t available. Legal teams that give themselves these options, by taking advantage of supportive technology, are better able to scale, strategize, and manage risk in the modern era of document review.ediscovery-review; ai-and-analyticsediscovery-review, ai-and-analytics, ai/big dataai-big-data eric pender
eDiscovery and Review
AI and Analytics
Blog
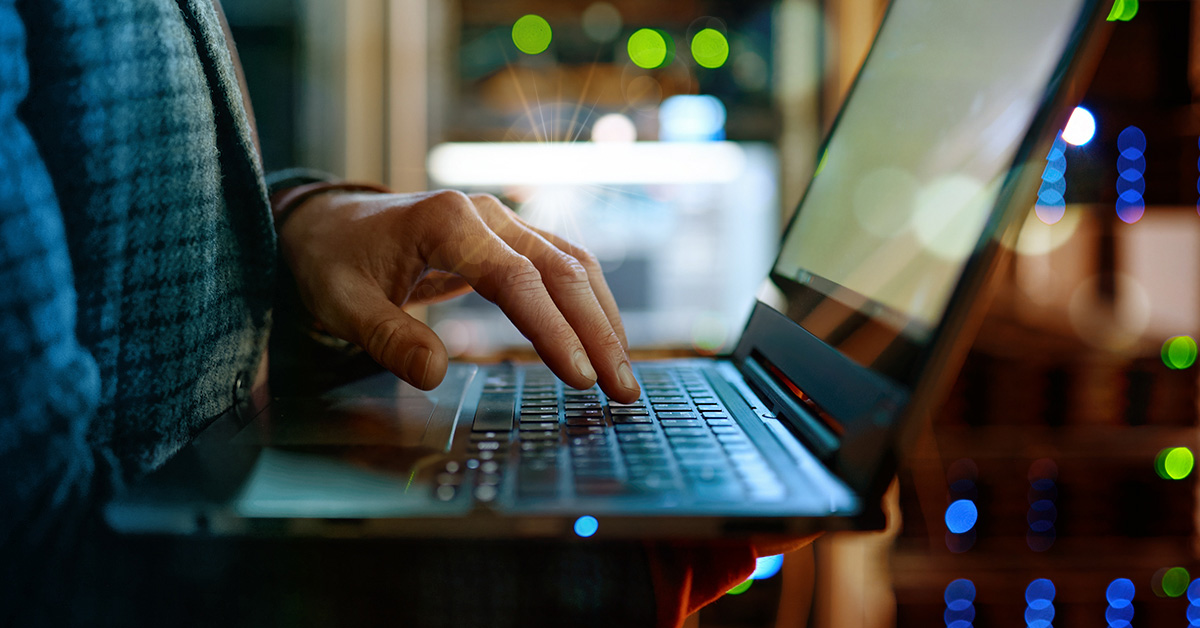
To Reduce Risk and Increase Efficiency in Investigations and Litigation, Data is Key
Handling large volumes of data during an investigation or litigation can be anxiety-inducing for legal teams. Corporate datasets can become a minefield of sensitive, privileged, and proprietary information that legal teams must identify as quickly as possible in order to mitigate risk. Ironically, corporate data also provides a key to speeding up and improving this process. By reusing metadata and work product from past matters in combination with advanced analytics, organizations can significantly reduce risk and increase efficiency during the review process.In a recent episode of Law & Candor, I discussed the complex nature of corporate data and ways in which the work done on past matters—coupled with analytics and advanced review tools—can be reused and leveraged to reduce risk and increase efficiency for current and future matters. Here are my key takeaways from the conversation.From burden to asset: leveraging data and analytics to gain the advantageThe evolution of analytical tools and technologies continues to change the data landscape for litigation and investigations. In complex matters especially—think multi-district litigation, second requests, large multi-year projects with multiple review streams—the technology and analytics that can now be applied to find responsive data not only helps streamline the review process but can extend corporate knowledge beyond a single matter for a larger purpose. Companies can now use their data to their advantage, transforming it from a liability into an asset. Prior to standardization around threading and TAR and CAL workflows, repository models were the norm. Re-use of issue coding was the best way to gain efficiency, but each matter still began with a clean slate. Now, with more sophisticated analytics, it’s not just coding and work product that can be re-used. The full analysis that went into making coding decisions can be applied to other matters so that the knowledge gained from a review and from the data itself is not lost as new matters come along. This results in greater overall efficiencies—not to mention major cost-savings—over time.Enhanced tools and analytics reduce the risk of PII, privilege, and other sensitive data exposureWith today’s data volumes, the more traditional methods used in review, such as search terms and regular expression (regex), can often result in high data recall with low precision. That is, such a wide net is cast that a lot of data is captured that isn’t terribly significant, and data that does matter can be missed. Analytical modeling can help avoid that pitfall by leveraging prior work product and coding to reduce the size of the data population from the outset, sometimes by as much as 90%, and to help find information that more traditional tools often miss.This is especially impactful when it comes to PII, PHI, and privileged or other sensitive data that may be in the population, because the risk of exposure is significantly reduced as accuracy increases. Upfront costs may seem like a barrier, but downstream cost savings in review make up for itWhen technology and data analytics are used to reduce data volume from the beginning, efficiencies are gained throughout the entire review process; there are exponential gains moving forward in terms of both speed and cost. Unfortunately, the upfront costs may seem steep to the uninitiated, presenting what is the likely barrier to the lack of wide adoption of many advanced technologies. The initial outlay before a project even begins can be perceived as a challenge for eDiscovery cost centers. Also, it can be very difficult for any company to keep up with the rapid evolution of both the complex data landscape and the analytics tools available to address it—the options can seem overwhelming. Finding the right technology partner with both expertise and experience in the appropriate analytics tools and workflows is crucial for making the transition to a more effective approach. A good partner should be able to understand the needs of your company and provide the necessary statistics to support and justify a change. A proof-of-concept exercise is a way to provide compelling evidence that any up-front expenditure will more than justify a revised workflow that will exponentially reduce costs of linear document review.How to get startedSeeing is believing, as they say, and the best way to demonstrate that something works is to see it in action. A proof-of-concept exercise with a real use case—run side-by-side with the existing process—is an effective way to highlight the efficiencies gained by applying the appropriate analytics tools in the right places. A good consulting partner, especially one familiar with the company’s data landscape, should be able to design such a test to show that the downstream cost savings will justify the up-front spend, not just for a single matter, but for other matters as well. Cross-matter analysis and analytics: the new frontierTAR and CAL workflows, which are finally finding wider use, should be the first line of exploration for companies not yet well-versed in how these workflows can optimize efficiency. But that is just the beginning. Advanced analytics tools add an additional level of robustness that can put those workflows into overdrive. Cross-matter analysis and analytics, for example, can address important questions: How can companies use the knowledge and work product gleaned from prior matters and apply them to current and future matters? How can such knowledge be pooled and leveraged, in conjunction with AI or other machine learning tools, to create models that will be applicable to future efforts?Marrying the old school data repository concept with new analytics tools is opening a new world of possibilities that we’re just beginning to explore. It’s a new frontier, and the most intrepid explorers will be the ones that reap the greatest benefits. For more information on data reuse and other review strategies, check out our review solutions page.ai-and-analytics; data-privacy; ediscovery-reviewcorporate; ai-and-analytics; analytics; big-data; compliance-and-investigations; corporationcassie blum
AI and Analytics
Data Privacy
eDiscovery and Review
Blog
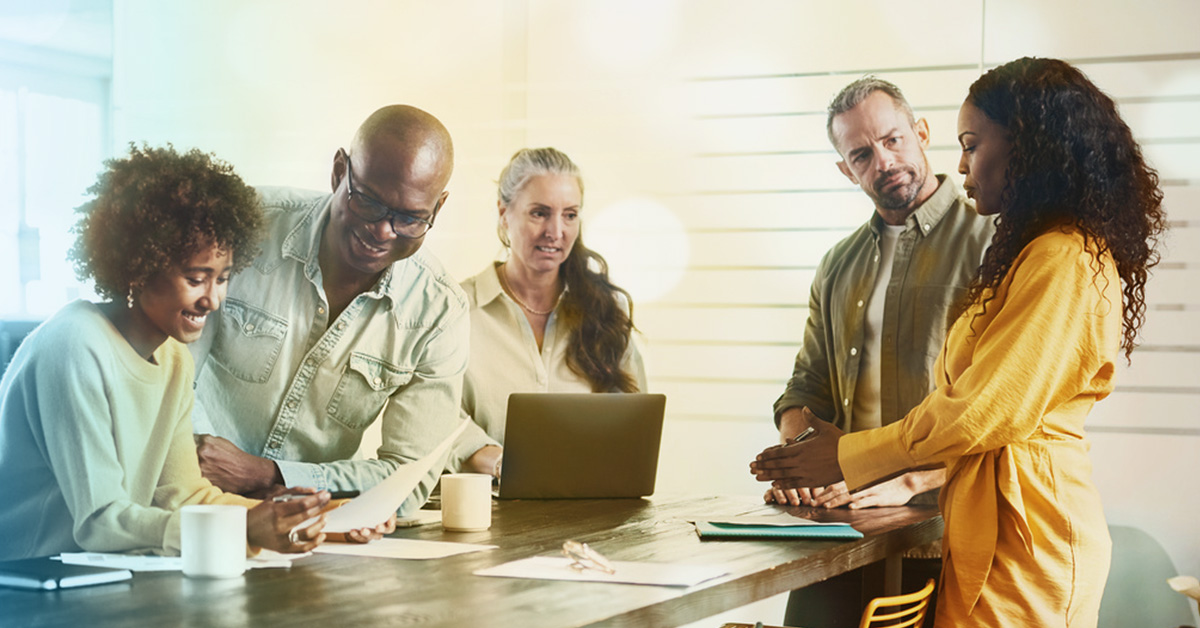
Three Ways to Use eDiscovery Technology to Reduce Repeated Review
By now, legal teams facing discovery are aware of many of the common technology and technology-enabled workflows used to increase the efficiency of document review on a single matter. But as data volumes grow and legal budgets shrink, legal teams must begin to think beyond a “matter-by-matter” approach. They must start applying technology more innovatively to create efficiencies across matters to minimize the burden of repeatedly reviewing the same documents again and again. Fortunately, many common technology-enabled review workflows (e.g., technology assisted review (TAR), advanced search guided by linguistic experts, and AI-powered review analytics) can help teams apply work product and insights from past matters to current and future matters. This not only saves time but also increases consistency and lowers the risk of inadvertent disclosures and cumbersome clawbacks.The opportunity to reduce repeated review is quite large, both because the problem is rampant and the technology that can help solve it is underutilized. A 2022 survey by the ABA showed that “predictive coding” is the least common application of eDiscovery software, used by only one in five law firms. In fact, 73% of respondents said they don’t know what predictive coding is (we explain it below). As document review continues to grow in complexity, and budget and other constraints apply pressure from other directions, more organizations should consider taking advantage of everything that technology has to offer.Repeated review is a large and familiar burdenRepeated review is baked into the status quo. Matters spanning multiple jurisdictions, civil litigations tied to government investigations, and matters involving the same or related IP are just a few examples in which the same documents could come up for review multiple times. Instead of looking across matters holistically, legal teams often feel obligated to roll up their sleeves, lower their heads, and review the same documents all over again—even when relevancy overlaps and for categories of information that remain relatively static across matters (privilege, trade secret, personally identifiable information (PII), etc.). This has obvious consequences for time and cost. The time invested on reviewing documents for privilege in a current matter, for example, becomes time saved on future matters involving those same documents. Risk is a factor as well. A document classified as privileged, or that contains PII or another sensitive category, in one matter should be classified the same way in the next one. But without a record of past matters, attorneys start over from scratch each time, which opens the door to inconsistency. And while it’s certainly possible to undo the mistake of producing sensitive documents, it can be quite time-consuming and expensive.Rejecting the status quo While the burden and risks associated with repeated review are felt every day, few legal teams and professionals are searching for a solution. Those willing to look beyond the status quo, however, will see that repeated review isn’t actually necessary, at least not to the degree that it’s done today. We also find that the keys to reducing repeated review lie in technology that many teams already use or have access to.Reusing work product from TAR and CAL workflows TAR 1.0, TAR 2.0, and Continuous Active Learning (CAL) workflows use machine learning technology to search and classify documents based on human input and their own ability to learn and recognize patterns. This is called predictive coding and it’s most often used to prioritize responsive documents for human review. The parameters for responsiveness change with the topics of each matter, so it’s not always possible to reuse those classifications on other matters. TAR and CAL tools can also be effective at making classifications around privilege, PII, and junk documents, which are not redefined from matter to matter. If a document was junk last time (say company logos attached to emails, blank attachments, etc.) it’s going to be junk this time too. Therefore, reusing these classifications made by technology on one matter can save legal teams even more time in the future. Refining review with linguistic expertsLinguistic experts add an extra layer of nuance to document review technology that makes them more precise and effective at classification. They develop complex criteria, based on intricate rules of syntax and language, to search and identify documents in a more targeted way than TAR and CAL tools.They can also help reduce repeated review by conducting bespoke searches informed by past matters. This process is more hands-on than using TAR and CAL tools; human linguists take lessons learned from one matter and incorporate them into their work on a related matter. It’s also more refined, so it can help in ways that TAR and CAL tools can’t.Litigation related to off-label drug use offers a good example. A company might have multiple matters tied to different drugs, making relevance unique for each matter. In this scenario, linguistics experts can identify linguistic markers that show how sales reps communicated with healthcare providers within that company. Then when the next off-label document review project begins, documents with those identifiers can be segregated for faster review. In this way, work from linguistic experts in one matter can help improve efficiency and minimize first-level review work on new matters. Apply learnings across matters using AI Review tools built on AI can reduce repeated review by classifying documents based on how they were classified before. AI tools can act as a “central mind” across matters, using past decisions on company data to make highly precise classifications on new matters. The more matters the AI is used on, the more precise its classifications become. The beauty here is that it applies to any amount of overlap across matters. The AI will recognize any documents that it has reviewed previously and will resurface their past classifications.Some AI tools can even retain the decision on past documents and associate it with a unique hash tag, so that it can tell reviewers how the same or similar documents were coded in previous matters—without the concern of over-retaining documents from past matters. Curious to challenge your status quo?TAR, AI, and other solutions can be invaluable parts of a legal team’s effort to curb repeated review — but they’re not the only part. In fact, the most important factor is a team’s mindset. It takes forethought and commitment to depart from the status quo, especially when it involves unfamiliar tools or strategies.The benefits can be profound, and the road to achieving them may be more accessible than you think.Find tips for starting small, as well as more information about how and why to address the burden of repeated review, in our deep dive on the subject.ai-and-analytics; ediscovery-review; lighting-the-path-to-better-ediscoveryreview, ai-big-data, blog, ai-and-analytics, ediscovery-reviewreview; ai-big-data; blogminimizing-re-reviewsarah moran
AI and Analytics
eDiscovery and Review
Lighting the Path to Better eDiscovery
No items found. Please try different search parameters.