
Table of Contents
AI for eDiscovery: Terminology to Know
Everybody’s talking about AI.
To help you follow the conversation, here’s a down-to-earth guide to artificial intelligence terminology and concepts with the most immediate impact on document review and eDiscovery.
Interact below or get the shareable infographic.
And to better understand how your peers are thinking about AI, we recently surveyed eDiscovery experts about how they're leveraging AI technology.
<h3 style="text-align: left;">Predictive AI</h3>
<h4 style="text-align: left;">AI that predicts what is true, now or in the future</h4>
<p style="text-align: left;">Give predictive AI lots of data—about the weather, human illness, the shows people choose to stream—and it will make predictions about what else might be true or might happen next. </p>
<p style="text-align: left;">These predictions are weighted by probability, which means predictive AI is concerned with the precision of its output.</p>
<h4 style="text-align: left;">Predictive AI in eDiscovery: Available now</h4>
<p style="text-align: left;">Tools with predictive AI use data from training sets and past matters to predict whether new documents fit the criteria for responsiveness, privilege, PII, and other classifications.</p>
<h3 style="text-align: left;">Generative AI</h3>
<h4 style="text-align: left;">AI that generates new content based on examples of existing content</h4>
<p style="text-align: left;">ChatGPT is a famous example. It was trained on massive amounts of written content on the internet. When you ask it a question, you’re asking it to generate more written content. </p>
<p style="text-align: left;">When it answers, it isn’t considering facts. It’s lining up words that it calculates will fulfill the request, without concern for precision.</p>
<h4 style="text-align: left;">Generative AI in eDiscovery: Still emerging</h4>
<p style="text-align: left;">So far, we have seen chatbots enter the market. Eventually it may take many forms, such as creating a first draft of eDiscovery deliverables based on prompts or prior inputs.</p>
<h5 style="text-align: left;">Predictive AI and Generative AI are types of </h5>
<h3 style="text-align: left;">Large Language Models (LLMs)</h3>
<h4 style="text-align: left;">AI that analyzes language in the ways people actually use it</h4>
<p style="text-align: left;">LLMs treat words as interconnected pieces of data whose meaning changes depending on the context. For example, an LLM recognizes that “train” means something different in the phrases “I have a train to catch” and “I need to train for the marathon.” </p>
<h4 style="text-align: left;">Large Language Models in eDiscovery: Available, but not universal</h4>
<p style="text-align: left;">Many document review tools and platforms use older forms of AI that aren’t built with LLMs. As a result, they miss the nuances of language and view every instance of a word like “train” equally.</p>
<h3 style="text-align: left;">Predictive AI</h3>
<h4 style="text-align: left;">AI that predicts what is true, now or in the future</h4>
<p style="text-align: left;">Give predictive AI lots of data—about the weather, human illness, the shows people choose to stream—and it will make predictions about what else might be true or might happen next. </p>
<p style="text-align: left;">These predictions are weighted by probability, which means predictive AI is concerned with the precision of its output.</p>
<h4 style="text-align: left;">Predictive AI in eDiscovery: Available now</h4>
<p style="text-align: left;">Tools with predictive AI use data from training sets and past matters to predict whether new documents fit the criteria for responsiveness, privilege, PII, and other classifications.</p>
<h3 style="text-align: left;">Generative AI</h3>
<h4 style="text-align: left;">AI that generates new content based on examples of existing content</h4>
<p style="text-align: left;">ChatGPT is a famous example. It was trained on massive amounts of written content on the internet. When you ask it a question, you’re asking it to generate more written content. </p>
<p style="text-align: left;">When it answers, it isn’t considering facts. It’s lining up words that it calculates will fulfill the request, without concern for precision.</p>
<h4 style="text-align: left;">Generative AI in eDiscovery: Still emerging</h4>
<p style="text-align: left;">So far, we have seen chatbots enter the market. Eventually it may take many forms, such as creating a first draft of eDiscovery deliverables based on prompts or prior inputs.</p>
<h5 style="text-align: left;">Predictive AI and Generative AI are types of </h5>
<h3 style="text-align: left;">Large Language Models (LLMs)</h3>
<h4 style="text-align: left;">AI that analyzes language in the ways people actually use it</h4>
<p style="text-align: left;">LLMs treat words as interconnected pieces of data whose meaning changes depending on the context. For example, an LLM recognizes that “train” means something different in the phrases “I have a train to catch” and “I need to train for the marathon.” </p>
<h4 style="text-align: left;">Large Language Models in eDiscovery: Available, but not universal</h4>
<p style="text-align: left;">Many document review tools and platforms use older forms of AI that aren’t built with LLMs. As a result, they miss the nuances of language and view every instance of a word like “train” equally.</p>
<h3 style="text-align: left;">Predictive AI</h3>
<h4 style="text-align: left;">AI that predicts what is true, now or in the future</h4>
<p style="text-align: left;">Give predictive AI lots of data—about the weather, human illness, the shows people choose to stream—and it will make predictions about what else might be true or might happen next. </p>
<p style="text-align: left;">These predictions are weighted by probability, which means predictive AI is concerned with the precision of its output.</p>
<h4 style="text-align: left;">Predictive AI in eDiscovery: Available now</h4>
<p style="text-align: left;">Tools with predictive AI use data from training sets and past matters to predict whether new documents fit the criteria for responsiveness, privilege, PII, and other classifications.</p>
<h3 style="text-align: left;">Predictive AI</h3>
<h4 style="text-align: left;">AI that predicts what is true, now or in the future</h4>
<p style="text-align: left;">Give predictive AI lots of data—about the weather, human illness, the shows people choose to stream—and it will make predictions about what else might be true or might happen next. </p>
<p style="text-align: left;">These predictions are weighted by probability, which means predictive AI is concerned with the precision of its output.</p>
<h4 style="text-align: left;">Predictive AI in eDiscovery: Available now</h4>
<p style="text-align: left;">Tools with predictive AI use data from training sets and past matters to predict whether new documents fit the criteria for responsiveness, privilege, PII, and other classifications.</p>
<h3 style="text-align: left;">Generative AI</h3>
<h4 style="text-align: left;">AI that generates new content based on examples of existing content</h4>
<p style="text-align: left;">ChatGPT is a famous example. It was trained on massive amounts of written content on the internet. When you ask it a question, you’re asking it to generate more written content. </p>
<p style="text-align: left;">When it answers, it isn’t considering facts. It’s lining up words that it calculates will fulfill the request, without concern for precision.</p>
<h4 style="text-align: left;">Generative AI in eDiscovery: Still emerging</h4>
<p style="text-align: left;">So far, we have seen chatbots enter the market. Eventually it may take many forms, such as creating a first draft of eDiscovery deliverables based on prompts or prior inputs.</p>
<h3 style="text-align: left;">Predictive AI</h3>
<h4 style="text-align: left;">AI that predicts what is true, now or in the future</h4>
<p style="text-align: left;">Give predictive AI lots of data—about the weather, human illness, the shows people choose to stream—and it will make predictions about what else might be true or might happen next. </p>
<p style="text-align: left;">These predictions are weighted by probability, which means predictive AI is concerned with the precision of its output.</p>
<h4 style="text-align: left;">Predictive AI in eDiscovery: Available now</h4>
<p style="text-align: left;">Tools with predictive AI use data from training sets and past matters to predict whether new documents fit the criteria for responsiveness, privilege, PII, and other classifications.</p>
<h3 style="text-align: left;">Predictive AI</h3>
<h4 style="text-align: left;">AI that predicts what is true, now or in the future</h4>
<p style="text-align: left;">Give predictive AI lots of data—about the weather, human illness, the shows people choose to stream—and it will make predictions about what else might be true or might happen next. </p>
<p style="text-align: left;">These predictions are weighted by probability, which means predictive AI is concerned with the precision of its output.</p>
<h4 style="text-align: left;">Predictive AI in eDiscovery: Available now</h4>
<p style="text-align: left;">Tools with predictive AI use data from training sets and past matters to predict whether new documents fit the criteria for responsiveness, privilege, PII, and other classifications.</p>
<h3 style="text-align: left;">Predictive AI</h3>
<h4 style="text-align: left;">AI that predicts what is true, now or in the future</h4>
<p style="text-align: left;">Give predictive AI lots of data—about the weather, human illness, the shows people choose to stream—and it will make predictions about what else might be true or might happen next. </p>
<p style="text-align: left;">These predictions are weighted by probability, which means predictive AI is concerned with the precision of its output.</p>
<h4 style="text-align: left;">Predictive AI in eDiscovery: Available now</h4>
<p style="text-align: left;">Tools with predictive AI use data from training sets and past matters to predict whether new documents fit the criteria for responsiveness, privilege, PII, and other classifications.</p>
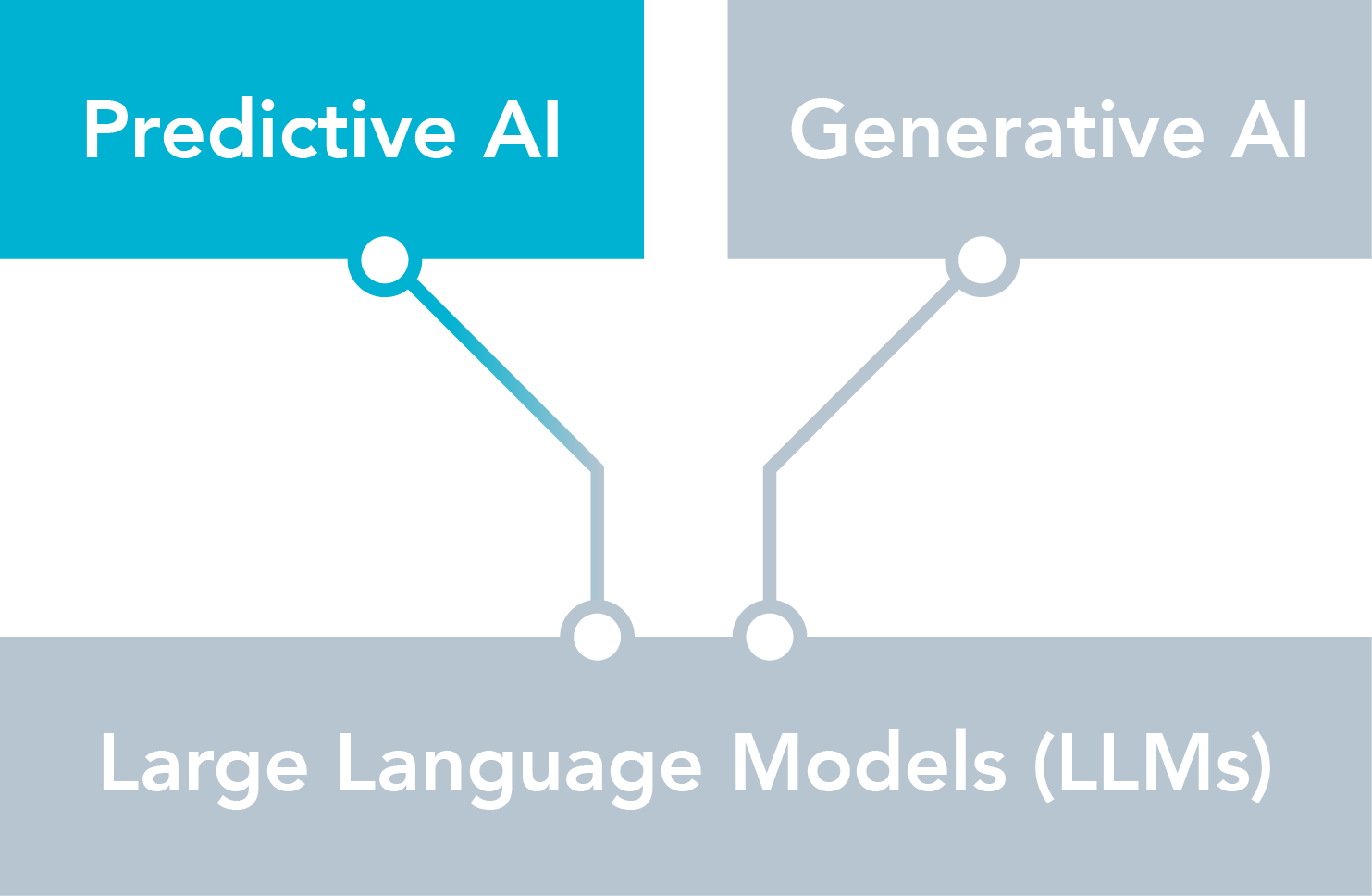
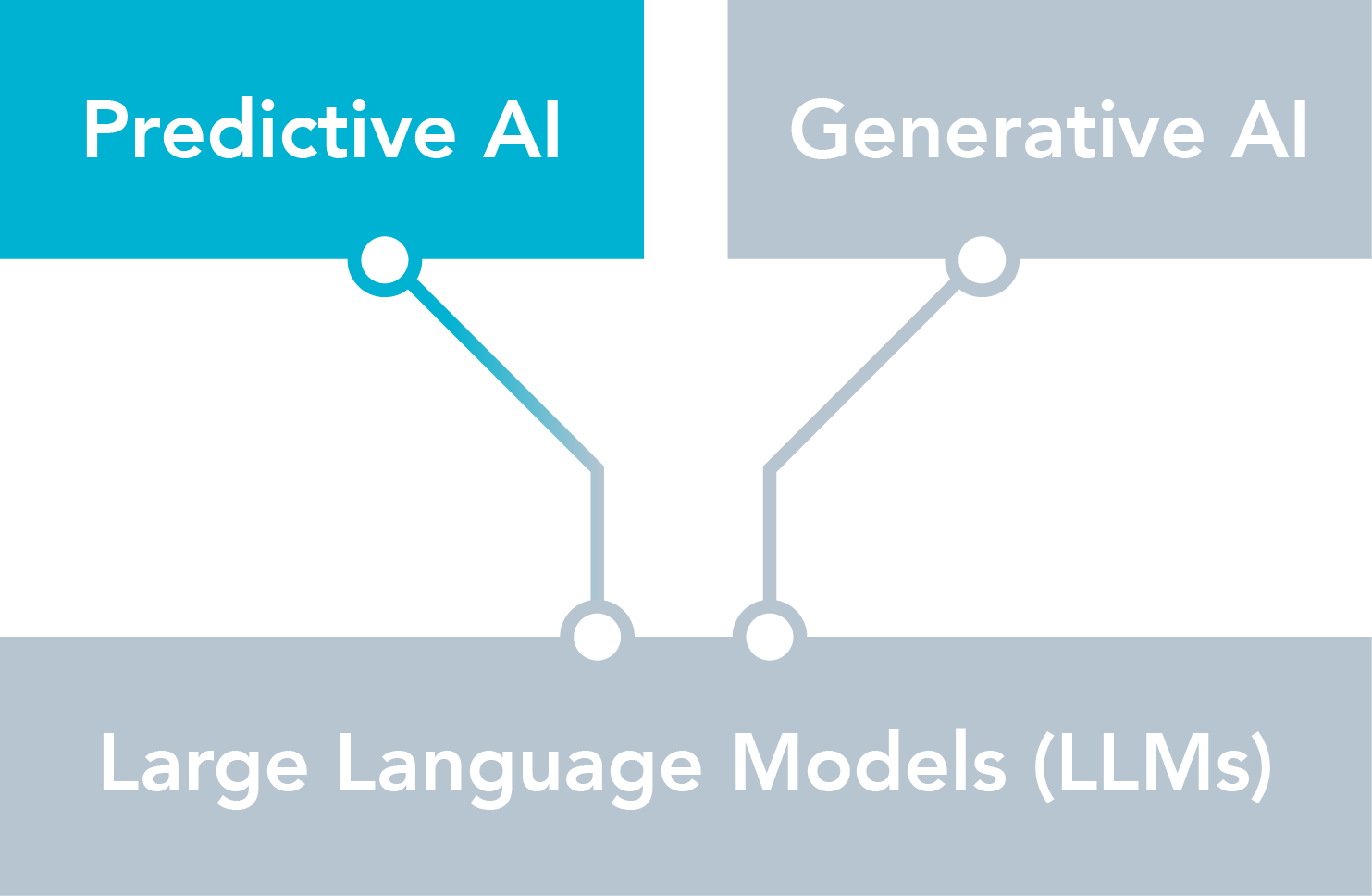
<h3 style="text-align: left;">Predictive AI</h3>
<h4 style="text-align: left;">AI that predicts what is true, now or in the future</h4>
<p style="text-align: left;">Give predictive AI lots of data—about the weather, human illness, the shows people choose to stream—and it will make predictions about what else might be true or might happen next. </p>
<p style="text-align: left;">These predictions are weighted by probability, which means predictive AI is concerned with the precision of its output.</p>
<h4 style="text-align: left;">Predictive AI in eDiscovery: Available now</h4>
<p style="text-align: left;">Tools with predictive AI use data from training sets and past matters to predict whether new documents fit the criteria for responsiveness, privilege, PII, and other classifications.</p>
<h3 style="text-align: left;">Predictive AI</h3>
<h4 style="text-align: left;">AI that predicts what is true, now or in the future</h4>
<p style="text-align: left;">Give predictive AI lots of data—about the weather, human illness, the shows people choose to stream—and it will make predictions about what else might be true or might happen next. </p>
<p style="text-align: left;">These predictions are weighted by probability, which means predictive AI is concerned with the precision of its output.</p>
<h4 style="text-align: left;">Predictive AI in eDiscovery: Available now</h4>
<p style="text-align: left;">Tools with predictive AI use data from training sets and past matters to predict whether new documents fit the criteria for responsiveness, privilege, PII, and other classifications.</p>
<h3 style="text-align: left;">Generative AI</h3>
<h4 style="text-align: left;">AI that generates new content based on examples of existing content</h4>
<p style="text-align: left;">ChatGPT is a famous example. It was trained on massive amounts of written content on the internet. When you ask it a question, you’re asking it to generate more written content. </p>
<p style="text-align: left;">When it answers, it isn’t considering facts. It’s lining up words that it calculates will fulfill the request, without concern for precision.</p>
<h4 style="text-align: left;">Generative AI in eDiscovery: Still emerging</h4>
<p style="text-align: left;">So far, we have seen chatbots enter the market. Eventually it may take many forms, such as creating a first draft of eDiscovery deliverables based on prompts or prior inputs.</p>
<h5 style="text-align: left;">Predictive AI and Generative AI are types of </h5>
<h3 style="text-align: left;">Large Language Models (LLMs)</h3>
<h4 style="text-align: left;">AI that analyzes language in the ways people actually use it</h4>
<p style="text-align: left;">LLMs treat words as interconnected pieces of data whose meaning changes depending on the context. For example, an LLM recognizes that “train” means something different in the phrases “I have a train to catch” and “I need to train for the marathon.” </p>
<h4 style="text-align: left;">Large Language Models in eDiscovery: Available, but not universal</h4>
<p style="text-align: left;">Many document review tools and platforms use older forms of AI that aren’t built with LLMs. As a result, they miss the nuances of language and view every instance of a word like “train” equally.</p>
<h3 style="text-align: left;">Predictive AI</h3>
<h4 style="text-align: left;">AI that predicts what is true, now or in the future</h4>
<p style="text-align: left;">Give predictive AI lots of data—about the weather, human illness, the shows people choose to stream—and it will make predictions about what else might be true or might happen next. </p>
<p style="text-align: left;">These predictions are weighted by probability, which means predictive AI is concerned with the precision of its output.</p>
<h4 style="text-align: left;">Predictive AI in eDiscovery: Available now</h4>
<p style="text-align: left;">Tools with predictive AI use data from training sets and past matters to predict whether new documents fit the criteria for responsiveness, privilege, PII, and other classifications.</p>
<h3 style="text-align: left;">Generative AI</h3>
<h4 style="text-align: left;">AI that generates new content based on examples of existing content</h4>
<p style="text-align: left;">ChatGPT is a famous example. It was trained on massive amounts of written content on the internet. When you ask it a question, you’re asking it to generate more written content. </p>
<p style="text-align: left;">When it answers, it isn’t considering facts. It’s lining up words that it calculates will fulfill the request, without concern for precision.</p>
<h4 style="text-align: left;">Generative AI in eDiscovery: Still emerging</h4>
<p style="text-align: left;">So far, we have seen chatbots enter the market. Eventually it may take many forms, such as creating a first draft of eDiscovery deliverables based on prompts or prior inputs.</p>
<h5 style="text-align: left;">Predictive AI and Generative AI are types of </h5>
<h3 style="text-align: left;">Large Language Models (LLMs)</h3>
<h4 style="text-align: left;">AI that analyzes language in the ways people actually use it</h4>
<p style="text-align: left;">LLMs treat words as interconnected pieces of data whose meaning changes depending on the context. For example, an LLM recognizes that “train” means something different in the phrases “I have a train to catch” and “I need to train for the marathon.” </p>
<h4 style="text-align: left;">Large Language Models in eDiscovery: Available, but not universal</h4>
<p style="text-align: left;">Many document review tools and platforms use older forms of AI that aren’t built with LLMs. As a result, they miss the nuances of language and view every instance of a word like “train” equally.</p>
<h3 style="text-align: left;">Predictive AI</h3>
<h4 style="text-align: left;">AI that predicts what is true, now or in the future</h4>
<p style="text-align: left;">Give predictive AI lots of data—about the weather, human illness, the shows people choose to stream—and it will make predictions about what else might be true or might happen next. </p>
<p style="text-align: left;">These predictions are weighted by probability, which means predictive AI is concerned with the precision of its output.</p>
<h4 style="text-align: left;">Predictive AI in eDiscovery: Available now</h4>
<p style="text-align: left;">Tools with predictive AI use data from training sets and past matters to predict whether new documents fit the criteria for responsiveness, privilege, PII, and other classifications.</p>
<h3 style="text-align: left;">Generative AI</h3>
<h4 style="text-align: left;">AI that generates new content based on examples of existing content</h4>
<p style="text-align: left;">ChatGPT is a famous example. It was trained on massive amounts of written content on the internet. When you ask it a question, you’re asking it to generate more written content. </p>
<p style="text-align: left;">When it answers, it isn’t considering facts. It’s lining up words that it calculates will fulfill the request, without concern for precision.</p>
<h4 style="text-align: left;">Generative AI in eDiscovery: Still emerging</h4>
<p style="text-align: left;">So far, we have seen chatbots enter the market. Eventually it may take many forms, such as creating a first draft of eDiscovery deliverables based on prompts or prior inputs.</p>
<h5 style="text-align: left;">Predictive AI and Generative AI are types of </h5>
<h3 style="text-align: left;">Large Language Models (LLMs)</h3>
<h4 style="text-align: left;">AI that analyzes language in the ways people actually use it</h4>
<p style="text-align: left;">LLMs treat words as interconnected pieces of data whose meaning changes depending on the context. For example, an LLM recognizes that “train” means something different in the phrases “I have a train to catch” and “I need to train for the marathon.” </p>
<h4 style="text-align: left;">Large Language Models in eDiscovery: Available, but not universal</h4>
<p style="text-align: left;">Many document review tools and platforms use older forms of AI that aren’t built with LLMs. As a result, they miss the nuances of language and view every instance of a word like “train” equally.</p>
Ask an Expert
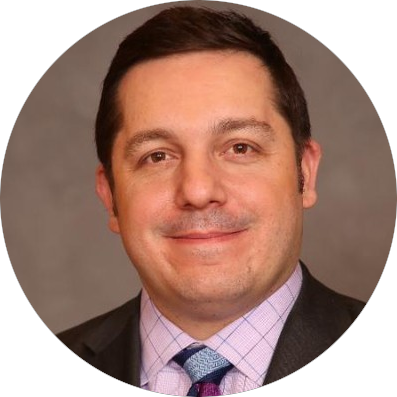
Karl Sobylak
Director of Product Management, Lighthouse
What about “hallucinations”?
This is a term for when generative AI produces written content that is false or nonsensical. The content may be grammatically correct, and the AI appears confident in what it’s saying. But the facts are all wrong. This can be humorous—but also quite damaging in legal scenarios.
Luckily, we can control and safeguard against this. Where defensibility is concerned, we can ensure that AI models provide the same solution every time. At Lighthouse, we always pair technology with skilled experts, who deploy QC workflows to ensure precision and high-quality work product.
What does this have to do with machine learning?
Machine learning is the older form of AI used by traditional TAR models and many review tools that claim to use AI. These aren’t built with LLMs, so they miss the nuance of language and view words at face value.
How does that compare to deep learning?
Deep learning is the stage of AI that evolved out of machine learning. It’s much more sophisticated, drawing many more connections between data. Deep learning is what enables the multi-layered analysis we see in LLMs.
For more expertise in using and governing AI effectively, check out AI at Lighthouse.
Ask an Expert
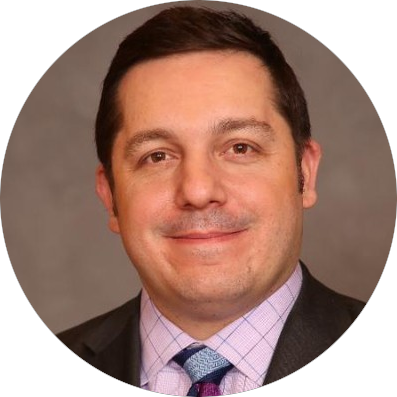
Karl Sobylak
Director of Product Management, Lighthouse
What about “hallucinations”?
This is a term for when generative AI produces written content that is false or nonsensical. The content may be grammatically correct, and the AI appears confident in what it’s saying. But the facts are all wrong. This can be humorous—but also quite damaging in legal scenarios.
Luckily, we can control and safeguard against this. Where defensibility is concerned, we can ensure that AI models provide the same solution every time. At Lighthouse, we always pair technology with skilled experts, who deploy QC workflows to ensure precision and high-quality work product.
What does this have to do with machine learning?
Machine learning is the older form of AI used by traditional TAR models and many review tools that claim to use AI. These aren’t built with LLMs, so they miss the nuance of language and view words at face value.
How does that compare to deep learning?
Deep learning is the stage of AI that evolved out of machine learning. It’s much more sophisticated, drawing many more connections between data. Deep learning is what enables the multi-layered analysis we see in LLMs.
For more expertise in using and governing AI effectively, check out AI at Lighthouse.
Ask an Expert
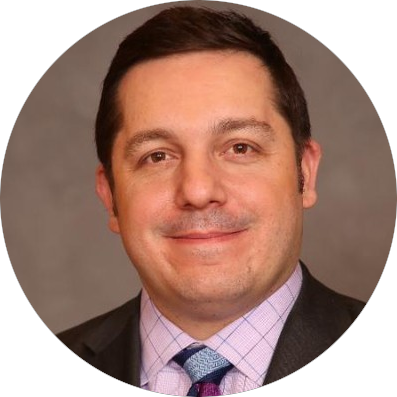
Karl Sobylak
Director of Product Management, Lighthouse
What about “hallucinations”?
This is a term for when generative AI produces written content that is false or nonsensical. The content may be grammatically correct, and the AI appears confident in what it’s saying. But the facts are all wrong. This can be humorous—but also quite damaging in legal scenarios.
Luckily, we can control and safeguard against this. Where defensibility is concerned, we can ensure that AI models provide the same solution every time. At Lighthouse, we always pair technology with skilled experts, who deploy QC workflows to ensure precision and high-quality work product.
What does this have to do with machine learning?
Machine learning is the older form of AI used by traditional TAR models and many review tools that claim to use AI. These aren’t built with LLMs, so they miss the nuance of language and view words at face value.
How does that compare to deep learning?
Deep learning is the stage of AI that evolved out of machine learning. It’s much more sophisticated, drawing many more connections between data. Deep learning is what enables the multi-layered analysis we see in LLMs.
For more expertise in using and governing AI effectively, check out AI at Lighthouse.
Ask an Expert
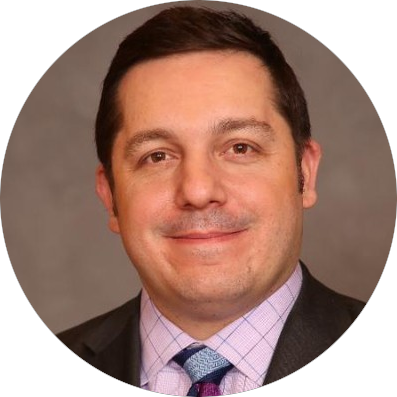
Karl Sobylak
Director of Product Management, Lighthouse
What about “hallucinations”?
This is a term for when generative AI produces written content that is false or nonsensical. The content may be grammatically correct, and the AI appears confident in what it’s saying. But the facts are all wrong. This can be humorous—but also quite damaging in legal scenarios.
Luckily, we can control and safeguard against this. Where defensibility is concerned, we can ensure that AI models provide the same solution every time. At Lighthouse, we always pair technology with skilled experts, who deploy QC workflows to ensure precision and high-quality work product.
What does this have to do with machine learning?
Machine learning is the older form of AI used by traditional TAR models and many review tools that claim to use AI. These aren’t built with LLMs, so they miss the nuance of language and view words at face value.
How does that compare to deep learning?
Deep learning is the stage of AI that evolved out of machine learning. It’s much more sophisticated, drawing many more connections between data. Deep learning is what enables the multi-layered analysis we see in LLMs.
For more expertise in using and governing AI effectively, check out AI at Lighthouse.
Ask an Expert
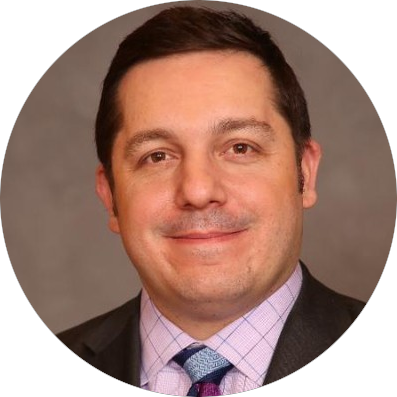
Karl Sobylak
Director of Product Management, Lighthouse
What about “hallucinations”?
This is a term for when generative AI produces written content that is false or nonsensical. The content may be grammatically correct, and the AI appears confident in what it’s saying. But the facts are all wrong. This can be humorous—but also quite damaging in legal scenarios.
Luckily, we can control and safeguard against this. Where defensibility is concerned, we can ensure that AI models provide the same solution every time. At Lighthouse, we always pair technology with skilled experts, who deploy QC workflows to ensure precision and high-quality work product.
What does this have to do with machine learning?
Machine learning is the older form of AI used by traditional TAR models and many review tools that claim to use AI. These aren’t built with LLMs, so they miss the nuance of language and view words at face value.
How does that compare to deep learning?
Deep learning is the stage of AI that evolved out of machine learning. It’s much more sophisticated, drawing many more connections between data. Deep learning is what enables the multi-layered analysis we see in LLMs.
For more expertise in using and governing AI effectively, check out AI at Lighthouse.
Ask an Expert
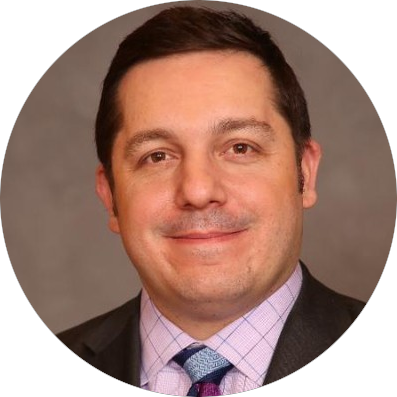
Karl Sobylak
Director of Product Management, Lighthouse
What about “hallucinations”?
This is a term for when generative AI produces written content that is false or nonsensical. The content may be grammatically correct, and the AI appears confident in what it’s saying. But the facts are all wrong. This can be humorous—but also quite damaging in legal scenarios.
Luckily, we can control and safeguard against this. Where defensibility is concerned, we can ensure that AI models provide the same solution every time. At Lighthouse, we always pair technology with skilled experts, who deploy QC workflows to ensure precision and high-quality work product.
What does this have to do with machine learning?
Machine learning is the older form of AI used by traditional TAR models and many review tools that claim to use AI. These aren’t built with LLMs, so they miss the nuance of language and view words at face value.
How does that compare to deep learning?
Deep learning is the stage of AI that evolved out of machine learning. It’s much more sophisticated, drawing many more connections between data. Deep learning is what enables the multi-layered analysis we see in LLMs.
For more expertise in using and governing AI effectively, check out AI at Lighthouse.
Ask an Expert
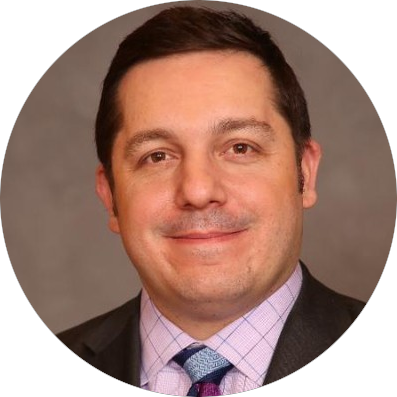
Karl Sobylak
Director of Product Management, Lighthouse
What about “hallucinations”?
This is a term for when generative AI produces written content that is false or nonsensical. The content may be grammatically correct, and the AI appears confident in what it’s saying. But the facts are all wrong. This can be humorous—but also quite damaging in legal scenarios.
Luckily, we can control and safeguard against this. Where defensibility is concerned, we can ensure that AI models provide the same solution every time. At Lighthouse, we always pair technology with skilled experts, who deploy QC workflows to ensure precision and high-quality work product.
What does this have to do with machine learning?
Machine learning is the older form of AI used by traditional TAR models and many review tools that claim to use AI. These aren’t built with LLMs, so they miss the nuance of language and view words at face value.
How does that compare to deep learning?
Deep learning is the stage of AI that evolved out of machine learning. It’s much more sophisticated, drawing many more connections between data. Deep learning is what enables the multi-layered analysis we see in LLMs.
For more expertise in using and governing AI effectively, check out AI at Lighthouse.
Ask an Expert
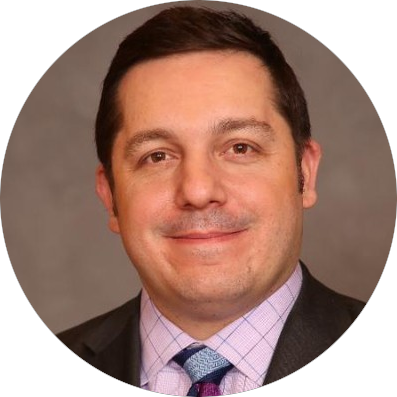
Karl Sobylak
Director of Product Management, Lighthouse
What about “hallucinations”?
This is a term for when generative AI produces written content that is false or nonsensical. The content may be grammatically correct, and the AI appears confident in what it’s saying. But the facts are all wrong. This can be humorous—but also quite damaging in legal scenarios.
Luckily, we can control and safeguard against this. Where defensibility is concerned, we can ensure that AI models provide the same solution every time. At Lighthouse, we always pair technology with skilled experts, who deploy QC workflows to ensure precision and high-quality work product.
What does this have to do with machine learning?
Machine learning is the older form of AI used by traditional TAR models and many review tools that claim to use AI. These aren’t built with LLMs, so they miss the nuance of language and view words at face value.
How does that compare to deep learning?
Deep learning is the stage of AI that evolved out of machine learning. It’s much more sophisticated, drawing many more connections between data. Deep learning is what enables the multi-layered analysis we see in LLMs.
For more expertise in using and governing AI effectively, check out AI at Lighthouse.
Ask an Expert
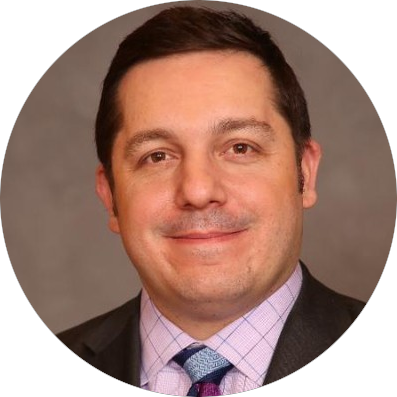
Karl Sobylak
Director of Product Management, Lighthouse
What about “hallucinations”?
This is a term for when generative AI produces written content that is false or nonsensical. The content may be grammatically correct, and the AI appears confident in what it’s saying. But the facts are all wrong. This can be humorous—but also quite damaging in legal scenarios.
Luckily, we can control and safeguard against this. Where defensibility is concerned, we can ensure that AI models provide the same solution every time. At Lighthouse, we always pair technology with skilled experts, who deploy QC workflows to ensure precision and high-quality work product.
What does this have to do with machine learning?
Machine learning is the older form of AI used by traditional TAR models and many review tools that claim to use AI. These aren’t built with LLMs, so they miss the nuance of language and view words at face value.
How does that compare to deep learning?
Deep learning is the stage of AI that evolved out of machine learning. It’s much more sophisticated, drawing many more connections between data. Deep learning is what enables the multi-layered analysis we see in LLMs.
For more expertise in using and governing AI effectively, check out AI at Lighthouse.
Ask an Expert
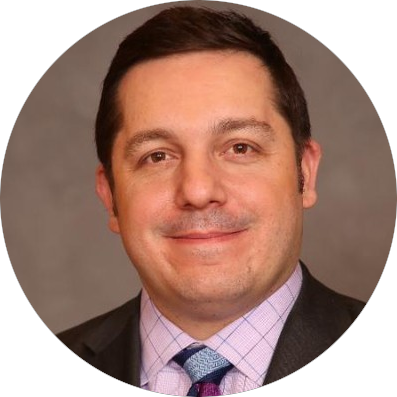
Karl Sobylak
Director of Product Management, Lighthouse
What about “hallucinations”?
This is a term for when generative AI produces written content that is false or nonsensical. The content may be grammatically correct, and the AI appears confident in what it’s saying. But the facts are all wrong. This can be humorous—but also quite damaging in legal scenarios.
Luckily, we can control and safeguard against this. Where defensibility is concerned, we can ensure that AI models provide the same solution every time. At Lighthouse, we always pair technology with skilled experts, who deploy QC workflows to ensure precision and high-quality work product.
What does this have to do with machine learning?
Machine learning is the older form of AI used by traditional TAR models and many review tools that claim to use AI. These aren’t built with LLMs, so they miss the nuance of language and view words at face value.
How does that compare to deep learning?
Deep learning is the stage of AI that evolved out of machine learning. It’s much more sophisticated, drawing many more connections between data. Deep learning is what enables the multi-layered analysis we see in LLMs.
For more expertise in using and governing AI effectively, check out AI at Lighthouse.
Ask an Expert
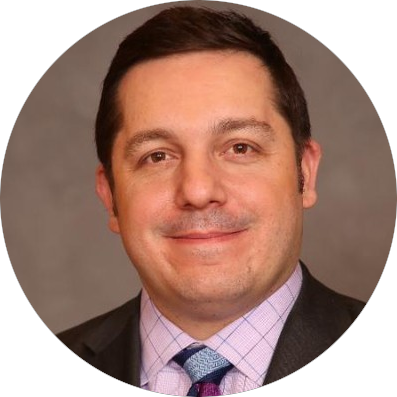
Karl Sobylak
Director of Product Management, Lighthouse
What about “hallucinations”?
This is a term for when generative AI produces written content that is false or nonsensical. The content may be grammatically correct, and the AI appears confident in what it’s saying. But the facts are all wrong. This can be humorous—but also quite damaging in legal scenarios.
Luckily, we can control and safeguard against this. Where defensibility is concerned, we can ensure that AI models provide the same solution every time. At Lighthouse, we always pair technology with skilled experts, who deploy QC workflows to ensure precision and high-quality work product.
What does this have to do with machine learning?
Machine learning is the older form of AI used by traditional TAR models and many review tools that claim to use AI. These aren’t built with LLMs, so they miss the nuance of language and view words at face value.
How does that compare to deep learning?
Deep learning is the stage of AI that evolved out of machine learning. It’s much more sophisticated, drawing many more connections between data. Deep learning is what enables the multi-layered analysis we see in LLMs.
For more expertise in using and governing AI effectively, check out AI at Lighthouse.
Subscribe to Lighthouse Insights
Get more like this and more delivered to your inbox.