Lighthouse Blog
Read the latest insights from industry experts on the rapidly evolving legal and technology landscapes with topics including strategic and technology-driven approaches to eDiscovery, innovation in artificial intelligence and analytics, modern data challenges, and more.
Thank you! Your submission has been received!
Oops! Something went wrong while submitting the form.
Thank you! Your submission has been received!
Oops! Something went wrong while submitting the form.
Blog

The Impact of Schrems II & Key Considerations for Companies Using M365: The Background
In 2016, European companies doing business in the US were able to breathe a sigh of relief. The European Commission deemed the Privacy Shield to be an adequate privacy protection. For the next half a decade, this shield, as well as Standard Contractual Clauses (SCCs), created the foundation upon which most global businesses were able to manage the thousands of data transfers that occur in each of their business days.Everything changed in July 2020 when the Court of Justice of the European Union gave its seismic judgment in a case generally known as Schrems II. As we will see, the decision has a particular impact on any companies relying on, or moving to, a cloud computing strategy. Businesses have been left needing to make a risk decision with seemingly no ideal outcome. Some legal, privacy, and compliance teams may be advocating for staying away from a cloud approach in light of the decision. The business teams, however, are focused on the vast array of benefits that cloud software offers.So what is the right decision? Where does the law stand and how do you manage your business in this uncertain time? In this four-part blog series, we&rsquo;ll explain the impact of Schrems II, provide practical tips for companies in the midst of making a cloud decision, give specific advice regarding companies who have, or are implementing, Microsoft&rsquo;s cloud offering (M365), and offer our view as to the future.Schrems II and Its ImpactFirst, let;s look at the Schrems II decision. The background to the case is well worth exploring but for the sake of brevity and providing actionable information we&rsquo;ll focus on the outcome and the consequences. The key outcomes impact the two primary ways in which most data transfers between Europe and the US:The EU-US Privacy Shield was invalidated with immediate effect.SCCs (the template contracts created by the EU Commission which are the most common way in which data is moved from the EU) were declared valid, but companies using SCCs could no longer just sign up and send. A company relying on SCCs would have to verify on a case-by-case basis that the personal data being transferred was adequately protected. This process is sometimes called a Transfer Impact Assessment, although the court did not coin that phrase. If the protection is inadequate, then additional safeguards could be needed.The consequences of the decision are still revealing themselves, but as things stand:The Privacy Shield (used by more than 5,000 mostly small-to-medium enterprises) has gone with no replacement in sight (although the Biden administration appears to recognise its importance with the rapid appointment of the experienced Christopher Hoff to oversee the process).There have been significant developments in relation to SCCs, additional safeguards, and transfer impact assessments:The US published a white paper to help organisations make the case that they should be able to send personal data to the US using approved transfer mechanisms.The European Data Protection Board (EDPB) published guidance on how to supplement transfer tools.The European Commission published draft replacement SCCs.The EDPB and the European Data Protection Supervisor adopted a joint opinion on the draft replacement SCCs requesting several amendments.There is not a clear timetable as to when the replacement SCCs or EDPB guidance (which has completed a period of publication consultation) will be finalised. The sooner the better because there seem to be inconsistencies between them. For example, the Schrems II judgment and draft replacement SCCs permit a risk assessment (i.e., it is possible to conclude that personal data might not be completely protected, but that the risk is so small that the parties can agree to proceed), whereas the EPDB recommendations seem to deal in black and white with no shades between (i.e., there is either adequate protection or there is not). It will be important to monitor which, if any, of these drafts moves and in which direction. Whether the SCCs are supported with a risk assessment or analysis along the lines of the EDPB recommendations (or perhaps both), going forward using SCCs may be rather cumbersome particularly in a cloud environment where the location and path of the data is not always crystal clear. Companies are therefore in something of a grey triangle, the sides of which are a judgment of the highest European Court, a draft replacement to the SCCs the Court reviewed in its judgment, and draft guidance about additional safeguards. In part two </span><span>of the series, we will offer companies some practical guidance on how to move forward in light of this grey triangle.To discuss this topic further, please feel free to reach out to us at info@lighthouseglobal.com.data-privacy; microsoft-365; information-governancemicrosoft, data-privacy, blog, privacy-shield, data-privacy, microsoft-365, information-governance,microsoft; data-privacy; blog; privacy-shieldlighthouse
Data Privacy
Microsoft 365
Information Governance
Blog

How Name Normalization Accelerates Privilege Review
A time-saving tool that consolidates different names for the same entity can make all the difference. One of the many challenges of electronic information and messaging rests in ascertaining the actual identity of the message creator or recipient. Even when only one name is associated with a specific document or communication, the identity journey may have only just begun.The many forms our monikers take as they weave in and out of the digital realm may hold no import for most exchanges, but they can be critical when it comes to eDiscovery and privilege review, where accurate identification of individuals and/or organizations is key.It’s difficult enough when common names are shared among many individuals (hello, John Smith?), but the compilation of our own singular name variations and aliases as they live in the realm of digital text and metadata make life no less complicated. In addition, the electronic format of names and email addresses as they appear in headers or other communications can also make a difference. Attempts to consolidate these variations when undertaking document review is painstaking and error-prone.Not metadata — people. Enter “name normalization.” Automated name normalization tools come to the rescue by isolating and consolidating information found in the top-level and sub-level email headers. Automated name normalization is designed to scan, identify, and associate the full set of name variants, aliases, and email addresses for any individual referenced in the data set, making it easier to review documents related to a particular individual during a responsive review.The mindset shift from email sender and recipient information as simply metadata to profiles of individuals is a subtle but compelling one, encouraging case teams and reviewers to consider people-centric ways to engage with data. This is especially helpful when it comes to identifying what may be—and just as importantly what is not—a potentially privileged communication.Early normalization of names can optimize the privilege workflow.When and how name normalization is done can make a big difference, especially when it comes to accelerating privilege review. Name normalization has historically been a process executed at the end of a review for the purpose of populating information into a privilege log or a names key. However, performing this analysis early in the workflow can be hugely beneficial.Normalizing names at the outset of review or during the pre-review stage as data is being processed enables a team to gain crucial intelligence about their data by identifying exactly who is included in the correspondence and what organizations they may be affiliated with. With a set of easy-to-decipher names to work with instead of a mix of full names, nicknames, initials without context, and other random information that may be even more confusing, reviewers don’t have to rely on guesswork to identify people of interest or those whose legally-affiliated or adversarial status may trigger (or break) a privilege call.Name normalization tools vary, and so do their benefits. Not all name normalization tools are created equal, so it is important to understand the features and benefits of the one being used. Ideally, the algorithm in use maximizes the display name and email address associations as well as the quality and legibility of normalized name values, with as little cleanup required as possible. Granular fielded output options, including top level and sub-header participants is also helpful, as are simple tools for categorizing normalized name entities based on their function, such as privilege actors (e.g., in-house counsel, outside counsel, legal agent) and privilege-breaking third parties (e.g., opposing counsel, government agencies). The ability to automatically identify and classify organizations as well as people (e.g., government agencies, educational institutions, etc.) is also a timesaver.Identification of privilege-breaking third parties is important: although some third parties are acting as agents of either the corporation or the law firms in ways that would not break privilege, others likely would. Knowing the difference can allow a team to triage their privilege review by either eliminating documents that include the privilege breakers from the review entirely, significantly reducing the potential privilege pile, or organizing the review with this likelihood in mind, helping to prevent any embarrassing privilege claims that could be rejected by the courts.Products with such features can provide better privilege identification than is currently the norm, resulting in less volume to manage for privilege log review work later on and curtailing the re-reviews that sometimes occur when new privilege actors or breakers come to light later in the workflow. This information enables a better understanding of any outside firms and attorneys that may not have been included in a list of initial privilege terms and assists in prioritizing the review of documents that include explicit or implied interaction with in-house or outside counsel.Other privilege review and logging optimizers. Other analytics features that can accelerate the privilege review process are coming on the scene as AI tools become more accepted for document review. Privilege Analytics from within Lighthouse Matter Analytics can help review teams with this challenging workflow, streamlining and prioritizing second pass review with pre-built classifiers to automate identification of law firms and legal concepts, tag and tier potentially privileged documents, detect privilege waivers, create privilege reasons, and much more.Interested in how Name Normalization works in Privilege Analytics? Let us show you!ediscovery-review; ai-and-analyticsblog, name-normalization, privilege-review, ediscovery-review, ai-and-analyticsblog; name-normalization; privilege-reviewlighthouse
eDiscovery and Review
AI and Analytics
Blog
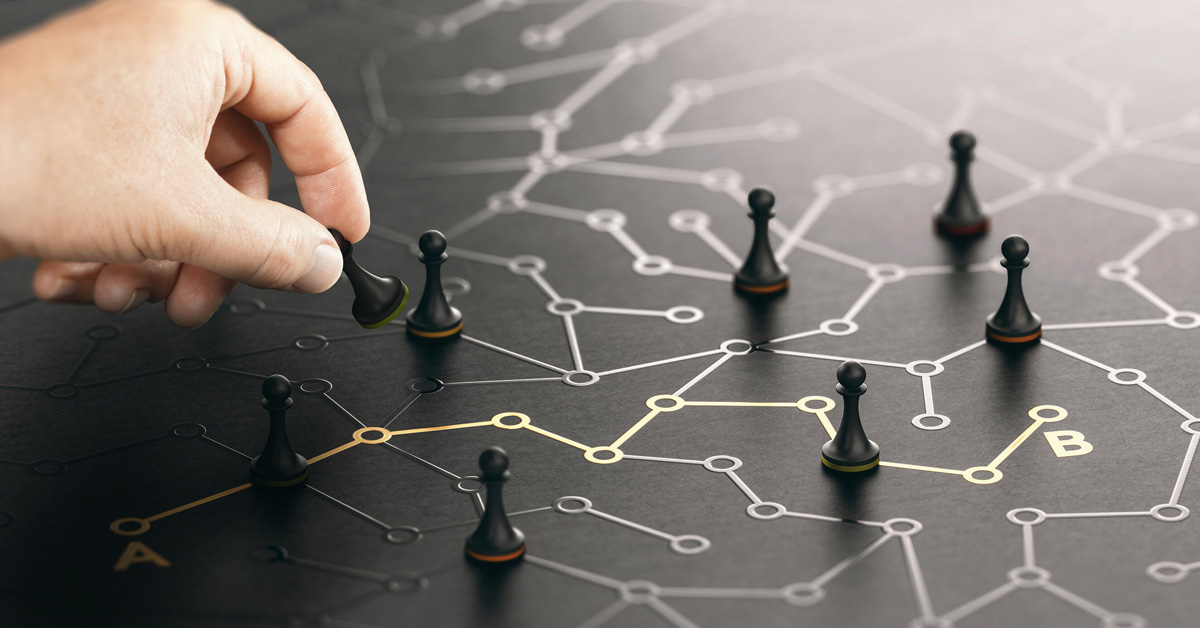
Legal Operations: From Tactical Resource to Strategic Partner
Do you ever feel like you are spending your day firefighting and wish you could spend more time planning and executing all the great ideas you have? Do you wish the business came to you first to ask for input so they could be prepared rather than rushing in once the alarm bells are already ringing? You are not alone. These are common refrains heard from legal operations professionals. Here are some ways to change that and go from a tactical resource to a strategic partner.Make Time for Strategic PlanningEven if the majority of your role calls for real-time execution, you can still showcase your strategic side. First, make sure you are spending time thinking strategically. I would recommend blocking out time at least once a month to do this work. During each of your thinking sessions, focus on just one idea. If you have too many ideas, your sessions will not be as productive. If you have multiple ideas you need to work through simultaneously, do so in multiple sessions. Or, if you don’t have any ideas, identify a need or frustration in your department. You can focus on a broad need (i.e. how to organize the department most efficiently) or a more narrow need (i.e. how to understand the company’s legal spend). When choosing what to focus on, choose something that you would be comfortable sharing with someone else. This will ensure that you can demonstrate the great strategic thinking you have done. Once you have selected the need you are going to think about, divide your time into three parts. Spend the first part brainstorming around all of the details of the specific need. Identify the problem and the potential causes. You can also identify related problems. Jot down the impact of the issue with as much detail as possible.In the second third, brainstorm potential solutions. Jot down anything that comes to mind. If this is an issue you have already thought about, you may even be able to identify how long each solution might take and/or the potential associated cost. If you have this information, note it. If not, that is ok too. The focus of the first two-thirds of your time should be to let the ideas flow. In the last third of your time, organize your thoughts on the first two sections. I find it easiest to do my organizing in a presentation software like PowerPoint, Google Slides, or Canva. You can follow the below outline or check out more details in my prior blog on getting your ideas approved at your organization.Problem Statement – Identify the issue in 1-3 sentences.Impact Statement – Identify the impact of the issue. You want to quantify this in some way, although at the early stages you might just put a placeholder or blank in here.Cause(s) – Identify the top 3-5 causes of the problem.Potential Solutions – How much you put here will really vary, but try to at least get your top idea into writing.Next Steps – Identify the next steps. If you’re not sure here, leave this blank. When you have your first conversation (more on that below), you can add information here. If you are clear about what you want to do, spend time on this section. This is an area where you can make any asks you have.Once you have completed your strategic thinking time, decide whether you want to share this plan. You may not do so with each monthly idea but you should share at least two outputs of your strategic thinking each year if you want to demonstrate your strategic ability. When you are sharing, I recommend starting with your boss. If that feels too vulnerable, you may decide to share with a co-worker first, but you will want to go to your boss next. Make sure you make clear that the goal of the session is to get their feedback. During that presentation, ask for feedback on the idea, next steps, as well as who else’s input might be valuable. If things go well, you will likely go forward with presenting to others. If your boss feels like this idea is not viable at this time, make sure you ask if there are any other similar projects that you can get involved in? Note that it might feel like a letdown if your boss says this isn’t the right time for this project. Keep in mind, however, that your goal was to showcase your strategic thinking and you will have accomplished this goal by presenting. I would also be remiss if I didn’t mention the primary obstacles I hear from people who “want” to do such an exercise. I don’t have time. I hear you – this is not something that is necessarily part of your day job, and if you’re fighting fires, you’re likely at your maximum capacity. However, think of this as a career investment. If you want to get out of the firefighting mode, invest in this work even if it is outside of your typical work hours or job responsibilities. I can’t take on the solution I’m suggesting. You can always have this discussion with your boss. It may be that there are resources that can help you or perhaps someone else takes on driving the solution. Either way, you will be able to showcase your strategic thinking.I’m worried that I will damage my reputation because this isn’t part of “my job.” Each organization is different and values this type of work differently. I will say that if this is something you really enjoy and is important to you, and your organization or role doesn’t value this work, you should consider whether your passions align with your current role.How to Show Up as Strategic in Tactical SituationsIf you can’t take on the strategic thinking right now, or if you want to press fast forward on you being seen as a strategic resource, there are ways you can show up strategically in your day-to-day interactions. When someone comes to you with a specific request for action, pause and ask yourself these three questions:“why” are we doing this;“what” broader impact will this have; and“how” does this relate to other things going on inside the organization? Take the example of a lawyer coming to you holding their latest law firm bill – fuming! “I just heard that we are paying twice on our matter for Firm ABC than Jane is paying on her matter for similar Firm ABD. Firm ABC’s rates are ridiculous – please negotiate them down right away.” You could absolutely pick up the phone and call Firm ABC. Or, you could think about the above questions. In doing so, you may realize that we are due for an annual firm rate adjustment across all our firms and that this firm has a very specialized area of expertise. If you share with this lawyer that the department has an overall rate discussion coming up that would potentially impact all of their matters, rather than just this one, as well as positively impact other matters with this firm. You can share that your preference would be to not make a phone call now but instead work this into a broader more strategic conversation with the firm. This second response showcases how you are thinking about the bigger picture and longer-term consequences for the organization. It also shares with the lawyer that you have proactive measures that you are working on that positively impact their world.legal-operationslegal-ops, blog, legal-operations,legal-ops; bloglighthouse
Legal Operations
Blog
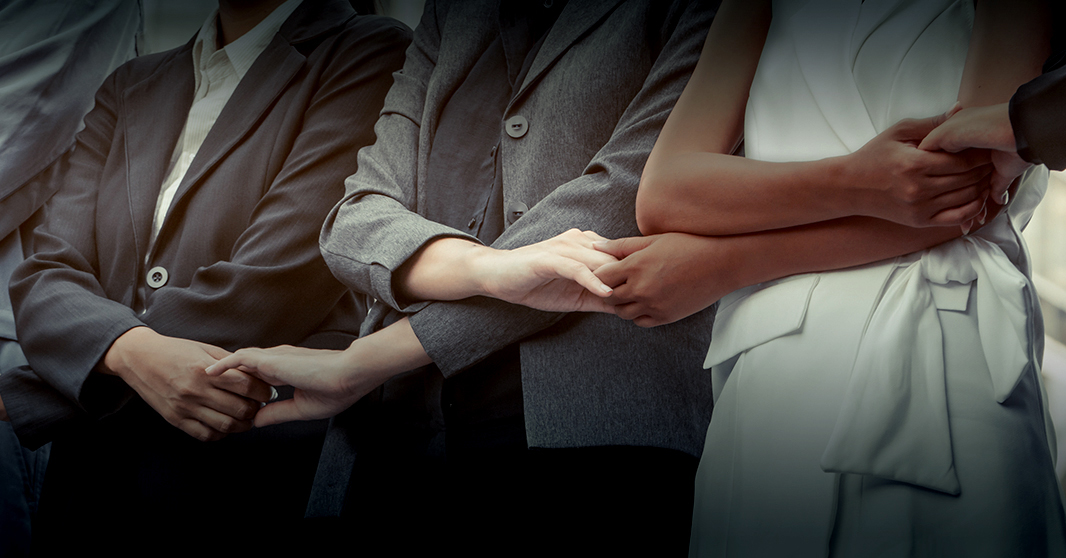
The Fearless 5: Spotlighting 5 Women Who “Choose to Challenge” Gender Bias in the Legal and Tech Fields
The International Women’s Day (IWD) theme for 2021 is “choose to challenge.”What a fitting theme for a year when 5.4 million women lost their jobs and over 2.1 million women left the workforce, while the COVID-19 pandemic wreaked havoc, and social and racial inequity issues were raised to the forefront of the international consciousness. There was certainly no shortage of challenges for women to choose from this past year.However, the IWD initiative website elaborates on the “choose to challenge” theme by noting that “a challenged world is an alert world and from challenge comes change.” It is that idea that should really resonate with us, as we move past 2020 and into 2021. There have been many examples this year of women who have chosen to rise to the challenges presented in 2020 and by doing so, have created change for other women.In keeping with this theme, Lighthouse is featuring five such women in the legal and technology fields. Five women who have risen to the challenges presented this year and created change. Those five women are:Laura Ewing-Pearle, eDiscovery Project Manager at Baker Botts LLPJenya Moshkovich, Assistant General Counsel at GenentechGina M. Sansone, Counsel – Litigation Support at Axinn Veltrop & Harkrider LLPAmy Sellars, Director, Discovery Center of Excellence at Cardinal HealthRebecca Sipowicz, VP and Assistant General Counsel at Ocwen Financial CorporationWe had the honor of interviewing these inspirational women about the “choose to challenge theme” – including the stressors of 2020, how to empower other women, how to leverage innovation to shape a more gender-equal world, and how to address social justice issues. That discussion led to some powerful lessons on how to rise to our current challenges and create meaningful, lasting change.Empowering Women Durning a PandemicLike any societal change, empowering women starts small, at the individual level. We do not have to wait for some grand opportunity or postpone our effort until we have the time to volunteer – especially during a pandemic when our personal time may feel even more precious and many in-person volunteer opportunities have halted. Empowerment can happen by simply reaching out to the women around us – women we work with, women in our personal lives, and women within our own families.Laura Ewing-Pearle noted, “One principle to keep in mind is that women are not a monolithic bloc, and that empowering women usually means empowering the individual. A woman with twenty years’ experience in the legal world caring for aging parents has a different set of stressors and goals than a woman fresh out of school with a toddler, especially under the new Covid protocols…Seeing past “woman” to the individual brings us all closer to a more gender-equal world.”In our professional lives, empowering at the individual level can mean reaching out to our women co-workers, teammates, and those that may be on “lower rungs” of the corporate or law firm ladders and offering them a chance to sit down (virtually) for a cup of coffee to talk about their personal goals and challenges. This provides women a space to be heard and seen, first and foremost. It is from these conversations that the seeds of change are often planted.Rebecca Sipowicz stated, “During the summer I became responsible for co-oversight of our back-office team in India, which is approximately 25% female. For at least the past 10 years, the India team has not reported, directly or indirectly, to a woman. I have reached out on an individual level to these women to discuss their career goals and how we can work together to achieve them. I also started a monthly “catch-up” where, in this virtual environment, we can meet for 30 minutes to talk about work, life, and the state of the world. Through these conversations, I have not only gotten to know better my colleagues who are located off-shore but also have been able to share life experiences, such as how to take advantage of our remote work world while parenting and managing online school. The continued growth of this group of employees is one of my most important goals for 2021.”These conversations often help us learn not only about individual ambitions and challenges, but also may help us learn about unsung accomplishments and milestones that women often are less apt to tout about themselves within their own organizations and networks. In turn, this can provide an excellent opportunity to be a champion for those women, by calling out successes that would otherwise go unrecognized.Gina Sansone said, “I am a strong believer in being a vocal cheerleader for the women around me who may be less comfortable with promoting their strengths and accomplishments. Women often play multiple roles at work and at home that are not obvious to others and get overlooked because they tend to be less measurable in a traditional sense. These contributions are nonetheless valuable and crucial to an organization and the lives of others, and it’s really important to notice and appreciate them along the way.”Empowering at the individual level also means leading by example during these conversations. The stressors of the pandemic have changed our lives dramatically, both professionally and personally. It is unchartered territory for everyone, and studies are showing that women are shouldering the brunt of the burden at home – often juggling full-time virtual schooling with children while working full-time jobs or dealing with the bulk of household maintenance. Leading by example and being honest about ourselves and our own hurdles during our conversations can empower women to be honest about their own struggles and needs.Jenya Moshkovich stated, “[I empower other women by] being honest about my own challenges and creating and holding space for others to be their true, authentic selves with all the complexities and messiness that can bring. The line between our private lives and work is blurrier now than it has ever been and we have to let go of trying to pretend that we have it all together all the time because no one does, especially these days.”The example we set and the honesty with which we portray ourselves can especially be important for those closest to us – the people within our own families and homes.Rebecca Sipowicz mentioned, “…Having my children home from school for six months enabled my 11-year-old daughter to witness firsthand how involved my job is and to learn how difficult but rewarding it is to juggle parenting and a career. This is a valuable lesson for all children, not just girls.”Leveraging Innovation to Shape a More Gender Equal WorldIf the legal and technology industries have anything in common, it is that women have been historically under-represented in both spaces. Fortunately, technological innovation can help close the gender gap in both industries:Rebecca Sipowicz shared, “The pandemic really pushed all of corporate America to take steps that will help to advance gender equality in the workplace – namely the move from in-office to remote work. This unquestionably provides working mothers with more equal access to the workplace. Through the use of video software such as Zoom and Teams, and the ability to work around parenting responsibilities, fewer women should feel the pressure to leave the workforce in order to parent….This flexibility allows women to continue to contribute to the workforce and grow in their careers while caring for their families, without feeling like they are short-changing either side. This should enable women to continue to take on more prominent roles and push women throughout the world to request an equal seat at the table.”Technological innovation can also help people push their organizations and law firms to empower women and support equality. Many companies have seen how innovation and technology can help close the gender gap, and we can work within those systems to further those efforts.Gina Sansone said, “I really don’t know how we can begin to work toward a gender-equal world without leveraging innovation. To me, innovation means creating a dynamic work environment that encourages everyone to move forward, which could mean training and managing members of the same team differently. While consistency is important, recognizing differences, being flexible, and empowering people to think differently and not simply check a box are steps toward shaping a more gender-equal world.”Laura Ewing-Pearle added, “Encouraging and leveraging more on-line training certainly helps anybody juggling family and career to keep pace with new technology and change. I’m grateful that Baker Botts as a firm encourages everyone to create new, innovative ideas to improve business processes and culture.”Jenya Moshkovich mentioned, “I am very fortunate to work for a company that has started innovating in this space years ago and where I am in the position to benefit from these efforts. Since 2007, Genentech has more than doubled the percentage of female officers from 16 to 43% and today over half of our employees and over half of our directors are women. In our legal department, ALL of our VPs are women. Genentech’s efforts to move towards gender equality have included senior leadership commitments, programs to drive professional development and open up opportunities for career advancement, among others.”Going forward, it will be equally important to continue efforts to support changes in our industry. While we have come a long way and made considerable progress, it is still important to push companies and law firms to recognize equity gaps and encourage the use of innovation and technology to help close those gaps.Amy Sellars stated, “Corporate practices favor men, and Covid exacerbates this problem. Will companies acknowledge that women took on most of the additional burdens of children at home, education at home, of people at home all the time (more dishes, more cleaning, more cooking, less dry cleaning, and more laundry)?”Rebecca Sipowicz said, “It is up to all of us to make sure that the realization that flexibility can result in increased productivity and satisfaction continues long after the pandemic, allowing women (and men of course) to have the best of both worlds.”Addressing Social Justice and Equality Issues2020 was also a devastating year for people of color, as well as underrepresented and low-income communities. The tragic events throughout the year brought social inequality issues and systemic racism to the forefront of the conversation in many families, workplaces, and social circles. Many of the lessons learned in the fight for gender equality can also be applied to the fight for racial and social equality. For instance, just as empowering women can start at the individual level, the fight for social and racial equality can also start with small, individual acts.These acts can be as simple as personally working to educate ourselves on the work to be done, so that we can act on social justice issues in the most impactful way:Jenya Moshkovich said, “2020 was a difficult year in so many ways including the tragic deaths of George Floyd, Breonna Taylor, and Ahmaud Arbery and many incidents of xenophobic violence against Asian Americans. My personal focus has been on educating myself, speaking up for others, listening, and fostering belonging. And there is so much more that needs to be done.”Gina Sansone added, “The social issues raised in 2020 were unfortunately just a magnification of issues that have existed for a long time. It was a perfect storm of events that certainly made me and others face the thought patterns, inequalities, and general civil unrest that has been festering in our society. It is very easy to live in a bubble and lose sight. I think one of the most important things that happened was people stopped being quiet and just accepting. Change will not happen unless it is absolutely forced and we need to continue recognizing that the world is not equal.”Creating social justice change also can mean utilizing the education we do have about these issues, and working within our communities to help in any way possible – both at the individual level and on a broader scale:Amy Sellars stated, “My husband and I have always been passionate about voting rights and participate in get out the vote efforts. 2020 was a particularly important year for voting issues, as so many people were isolated and had even less access to register to vote or get to polls than normal. Working with the League of Women Voters, we did neighborhood registration drives, and we volunteered as non-partisan poll watchers. We also picked up Meals on Wheels shifts. All around the country, meal recipients who used to be fed at central locations were transitioned to home deliveries, and it has taken an army of volunteers in personal vehicles…We are also volunteering on the domestic crisis hotline.”We can also leverage the networks and programs put in place within forward-thinking organizations to help bring about social change. More and more law firms and organizations are working to help close the gender gap and fight racial and social inequity. Employees of those organizations are in a unique position to join those initiatives to make more of an impact:Laura Ewing-Pearle said, “While (Baker Botts) had resources in place prior to the events of last year, the firm has also increased efforts over the past twelve months to address social issues including greater outreach to diverse communities, and creating a significant pro bono partnership with Official Black Wall Street, among other major initiatives.”Rebecca Sipowicz added, “I am a member of the Ocwen Global Women’s Network (OGWN), which supports the attainment of Ocwen’s goals in diversity, inclusion, and talent development. I am also on the planning committee for the National Association of Women Lawyers (NAWL) mid-year meeting. NAWL’s mission is to empower women in the legal profession, while cultivating a diverse membership dedicated to equality, mutual support and collective success. Membership in and support of organizations such as NAWL and OGWN provide me with a platform to address the diversity and social issues that permeated 2020.”‍ConclusionThe lesson learned from these strong women during a year full of challenges is that seemingly “small” acts can have big impacts. Change starts with all of us, at an individual level, working to empower women and make impactful societal changes – one person, one organization, and one community at a time.Thank you to the five women who participated in our 2021 International Women's Day Campaign! Take a look at our 2020 and 2019 International Women's Day campaigns for more inspiring stories of women in our industry making bold moves to promote gender equality.For more information, please reach out to us at info@lighthouseglobal.com.diversity-equity-and-inclusionblog, diversity-equity-and-inclusion,bloglighthouse
Diversity, Equity, and Inclusion
Blog

Now Live! Reed Smith's M365 in 5 Podcast Series
Lighthouse Microsoft 365 (M365) experts, John Holliday and John Collins, recently teamed up with Reed Smith to present the M365 in 5 Foundation Series on Reed Smith’s Tech Law Talks podcast. The series dives into operational considerations when rolling out M365 tools related to governance, retention, eDiscovery, and data security across a broad range of applications, from Exchange and SharePoint to all things Microsoft Teams.Check out the lineup below and click the titles of each podcast to give them a listen.M365 in 5 – Part 1: Exchange Online – Not just a mailboxDiscover the enhanced functionality of EXO, including new data types and the potential for enhanced governance.M365 in 5 – Part 2: SharePoint Online – The new file-share environmentHear about the enhanced file share and collaboration functionality in SharePoint Online, including real-time collaboration, access controls, and opportunities to control retention and deletion.M365 in 5 – Part 3: OneDrive for Business – Protected personal collaborationLearn about OneDrive for Business and how organizations can use it for personal document storage, such as giving other users access to individual documents within an individual’s OneDrive and acting as the storage location for all Teams Chats.M365 in 5 – Part 4: Teams – An introduction to collaborationListen to an introduction to Teams and how it is transforming the way organizations are working and communicating.M365 in 5 – Part 5: Teams Chats – Modern communicationsUncover the enhanced functionality of M365’s new instant messaging platform, including persistent chats, modern attachments, expressive features, and priority messaging, which enhance communication but can bring increased eDiscovery or regulatory risks.M365 in 5 – Part 6: Teams Channels – The virtual collaboration workspaceHear how Teams Channels are changing not only the way organizations work and collaborate, but also key legal and risk considerations that should be contemplated.M365 in 5 – Part 7: Teams Audio/Video (A/V) ConferencingDive into the functionality and controls of audio/video conferencing capabilities, including the integration of chats, whiteboards, translation, and transcription services.The Tech Law Talks podcast hosts regular discussions about the legal and business issues around data protection, privacy and security; data risk management; intellectual property; social media; and other types of information technology. For more information regarding the show, follow the link here: https://reedsmithtech.podbean.com.If you have questions about how to develop and maintain legal and compliance programs around M365, reach out to us at info@lighthouseglobal.com.microsoft-365; information-governancemicrosoft, blog, microsoft-365, information-governancemicrosoft; bloglighthouse
Microsoft 365
Information Governance
Blog

Mitigating eDiscovery Risk of Collaboration Tools
Below is a copy of a featured article written by Kimberly Quan of Juniper Networks and John Del Piero of Lighthouse for Bloomberg Law.Whether it's Teams, Slack, Zendesk, GChat, ServiceNow, or similar solutions that have popped up in the market over the last few years, collaboration and workflow platforms have arrived. According to Bloomberg Law's 2020 Legal Technology Survey, collaboration tools are being used by 77% of in-house and 44% of law firm attorneys. These tools are even more widely used by workers outside of the legal field.With many companies planning to make remote working a permanent fixture, we can expect the existing collaboration tools to become even more entrenched and new competitors to arrive on the scene with similarly disruptive technologies.This will be a double-edged sword for compliance and in-house legal teams, who want to encourage technology that improves employee productivity, but are also wary of the potential information governance and eDiscovery risks arising because of these new data sources. This article explains the risks these tools can pose to organizations and provides a three-step approach to help mitigate those risks.Understand Litigation and Investigation RiskThe colloquial and informal nature of collaborative tools creates inherent risk to organizations, much like the move from formal memos to email did 20 years ago. Communications that once occurred orally in the office or over the phone are now written and tracked, logged, and potentially discoverable. However, a corporation's ability to retain, preserve, and collect these materials may be unknown or impossible, depending on the initial licensing structure the employee or the company has entered into or the fact that many new tools do not include features to support data retention, preservation, or collection.Government agencies and plaintiffs’ firms have an eye on these new applications and platforms and will ask specifically about how companies and even individual custodians use them during investigations and litigations. Rest assured that if a custodian indicates during an interview or deposition that she used the chat function in a tool like Teams or Slack, for example, to work on issues relevant to the litigation, opposing counsel will ask for those chat records in discovery. Organizations can mitigate the risk of falling down on their eDiscovery obligations because of the challenges posed bycollaboration tool data using this three-step approach:Designate personnel in information technology (IT) and legal departments to work together to vet platforms and providers.Develop clear policies that are regularly reviewed for necessary updates and communicated to the platform users.Ensure internal or external resources are in place to monitor the changes in the tools and manage associated retention, collection, and downstream eDiscovery issues.Each of these steps is outlined further below.Designate IT & Legal Personnel to Vet Platforms and Providers‍Workers, especially those in the tech industry, naturally want to be free to use whatever technology allows them to effectively collaborate on projects and quickly share information.However, many of these tools were not designed with legal or eDiscovery tasks in mind, and therefore can pose challenges around the retention, preservation and collection of the data they generate.Companies must carefully vet the business case for any new collaboration tool before it is deployed. This vetting process should entail much more than simply evaluating how well the tool or platform can facilitate communication and collaboration between workers. It also involves designating personnel from both legal and IT to work together to evaluate the eDiscovery and compliance risks a new tool may pose to an organization before it is deployed.The importance of having personnel from both legal and IT involved from the outset cannot be understated. These two teams have different sets of priorities and can evaluate eDiscovery risks from two different vantage points. Bringing them together to vet a new collaboration tool prior to deployment will help to ensure that all information governance and eDiscovery downstream effects are considered and that any risks taken are deliberate and understood by the organization in advance of deployment. This collaborative team can also ensure that preservation and discovery workflows are tested and in place before employees begin using the tool.Once established, this dedicated collaborative IT and legal team can continue to serve the organization by meeting regularly to stay abreast of any looming legal and compliance risks related to data generation. For example, this type of team can also evaluate the risks around planned organizational technology changes, such as cloud migrations, or develop workflows to deal with the ramifications of the near-constant stream of updates that roll out automatically for most cloud-based collaborative tools.Develop Clear Policies That Are Regularly Reviewed‍The number of collaborative platforms that exist in the market is ever evolving, and it is tempting for organizations to allow employees to use whatever tool makes their work the easiest. But, as shown above, allowing employees to use tools that have not been properly vetted can create substantial eDiscovery and compliance risks for the organization.Companies must develop clear policies around employee use of collaborative platforms in order to mitigate those risks. Organizations have different capabilities in restricting user access to these types of platforms. Historically, technology companies have embraced a culture where innovation is more important than limiting employees’ access to the latest technology. More regulated companies, like pharmaceuticals, financial services, and energy companies, have tended to create a more restrictive environment. One of the most successful approaches, no matter the environment or industry, is to establish policies that restrict implementation of new tools while still providing users an avenue to get a technology approved for corporate use after appropriate vetting.These policies should have clear language around the use of collaboration and messaging tools and should be frequently communicated to all employees. They should also be written using language that does not require updating every time anew tool or application is launched on the market. For instance, a policy that restricts the work-related use of a broad category of messaging tools, like ephemeral messaging applications, also known as self-destructing messaging applications, is more effective than a policy that restricts the use of a specific application, like Snapchat. The popularity of messaging tools can change every few months, quickly leading to outdated and ineffective policies if the right language is not used.Make sure employees not only understand the policy, but also understand why the policy is in place. Explain the security, compliance, and litigation-related risks certain types of applications pose to the organization and encourage employees to reach out with questions or before using a new type of technology.Further, as always with any policy, consider how to audit and police its compliance. Having a policy that isn't enforced issometimes worse than having no policy at all.Implement Resources to Manage Changes in Tools‍Most collaboration tools are cloud-based, meaning technology updates can roll out on a near-constant basis. Small updates and changes may roll out weekly, while large systemic updates may roll out less frequently but include hundreds of changes and updates. These changes may pose security, collection, and review challenges, and can leave legal teams unprepared to respond to preservation and production requests from government agencies or opposing counsel. In addition, this can make third-party tools on which companies currently rely for specific retention and collection methodologies obsolete overnight.For example, an update that changes the process for permissions and access to channels and chats on a collaborative platform like Teams may seem like a minor modification. However, if this type of update is rolled out without legal and IT team awareness, it may mean that employees who formerly didn't have access to a certain chat function may now be able to generate discoverable data without any mechanism for preservation or collection in place.The risks these updates pose mean that is imperative for organizations to have a framework in place to monitor and manage cloud-based updates and changes. How that framework looks will depend on the size of the organization and the expertise and resources it has on hand. Some organizations will have the resources to create a team solely dedicated to monitoring updates and evaluating the impact of those updates. Other organizations with limited internal access to the type of expertise required or those that cannot dedicate the resources required for this task may find that the best approach is to hire an external vendor that can perform this duty for the organization.When confronted with the need to collect, process, review, and produce data from collaboration tools due to an impending litigation or investigation, an organization may find it beneficial to partner with someone with the expertise to handle the challenges these types of tools present during those processes. Full-scale, cloud-based collaboration tools like Microsoft Teams and Slack are fantastic for workers because of their ability to combine almost every aspect of work into a single, integrated interface. Chat messaging, conference calling, calendar scheduling, and group document editing are all at your fingertips and interconnected within one application. However, this aspect is precisely why these tools can be difficult to collect, review, and produce from an eDiscovery perspective.With platforms like Teams, several underlying applications, such as chat, video calls, and calendars, are now tied together through a backend of databases and repositories. This makes a seemingly simple task like “produce by custodian” or “review a conversation thread” relatively difficult if you haven't prepared or are not equipped to do so. For example, in Teams communications such as chat or channel messages, when a user sends a file to another user, the document that is attached to the message is no longer the static, stand-alone file.Rather, it is a modern attachment, a link to the document that resides in the sender's OneDrive. This can beg questions as to which version was reviewed by whom and when it was reviewed. Careful consideration of versioning and all metadata and properties will be of the utmost importance during this process, and will require someone on board who understands the infrastructure and implications of those functions.The type of knowledge required to effectively handle collection and production of data generated by the specific tools an organization uses will be extremely important to the success of any litigation or investigation. Organizations can begin planning for success by proactively seeking out eDiscovery vendors and counsel that have experience and expertise handling the specific type of collaboration tools that the organization currently uses or is planning on deploying. Once selected, these external experts can be engaged early, prior to any litigation or investigation, to ensure that eDiscovery workflows are in place and tested long before any production deadlines.ConclusionCloud-based collaboration tools and platforms are here to stay. Their ability to allow employees to communicate and collaborate in real time while working in a remote environment is becoming increasingly important in today's world. However, these tools inherently present eDiscovery risks and challenges for which organizations must carefully prepare. This preparation includes properly vetting collaboration tools and platforms prior to deploying them, developing and enforcing clear internal policies around their use, monitoring all system updates and changes, and engaging eDiscovery experts early in the process.With proper planning, good collaboration between IT and legal teams and expert engagement, organizations can mitigate the eDiscovery risks posed by these tools while still allowing employees the ability to use the collaboration tools that enable them to achieve their best work.Reproduced with permission. Published March 2021. Copyright © 2021 The Bureau of National Affairs, Inc.800.372.1033. For further use, please contact permissions@bloombergindustry.com.chat-and-collaboration-data; ediscovery-review; microsoft-365emerging-data-sources, blog, corporate, chat-and-collaboration-data, ediscovery-review, microsoft-365,emerging-data-sources; blog; corporatebloomberg law
Chat and Collaboration Data
eDiscovery and Review
Microsoft 365
Blog

AI and Analytics: New Ways to Guard Personal Information
Big data can mean big problems in the ediscovery and compliance world – and those problems can be exponentially more complicated when personal data is involved. Sifting through terabytes of data to ensure that all personal information is identified and protected is becoming an increasingly more painstaking and costly process for attorneys today.Fortunately, advances in artificial intelligence (AI) and analytics technology are changing the landscape and enabling more efficient and accurate detection of personal information within data. Recently, I was fortunate enough to gather a panel of experts together to discuss how AI is enabling legal professionals in the ediscovery, information governance, and compliance arenas to identify personal protected information (PII) and personal health information (PHI) more quickly within large datasets. Below is a summary of our discussion, along with some helpful tips for leveraging AI to detect personal information.Current Methods of Personal Data Identification Similar to the slower adoption of AI and analytics to help with the protection of attorney-client privilege information (compared to the broader adoption of machine learning to identify matter relevant documents), the legal profession has also been slow to leverage technology to help identify and protect personal data. Thus, the identification of personal data remains a very manual and reactive process, where legal professionals review documents one-by-one on each new matter or investigation to find personal information that must be protected from disclosure.This process can be especially burdensome for pharmaceutical and healthcare industries, as there is often much more personal information within the data generated by those organizations, while the risk for failing to protect that information may be higher due to healthcare-specific patient privacy regulations like HIPAA.How Advances in AI Technology Can Improve Personal Data Identification There are a few ways in which AI has advanced over the last few years that make new technology much more effective at identifying personal data:Analyzing More Than Text: AI technology is now capable of analyzing more than just the simple text of a document. It can now also analyze patterns in metadata and other properties of documents, like participants, participant accounts, and domain names. This results in technology that is much more accurate and efficient at identifying data more likely to contain personal information.Leveraging Past Work Product: Newer technology can now also pull in and analyze the coding applied on previous reviews without disrupting workflows in the current matter. This can add incredible efficiency, as documents previously flagged or redacted for personal information can be quickly removed from personal information identification workflows, thus reducing the need for human review. The technology can also help further reduce the amount of attorney review needed at the outset of each matter, as it can use many examples of past work product to train the algorithms (rather than training a model from scratch based on review work in the current matter).Taking Context into Account: Newer technology can now also perform a more complicated analysis of text through algorithms that can better assess the context of a document. For example, advances in Natural Language Processing (NLP) and machine learning can now identify the context in which personal data is often communicated, which helps eliminate previously common false hits like mistakenly flagging phone numbers as social security numbers, etc.Benefits of Leveraging AI and Analytics when Detecting Sensitive DataArguably the biggest benefit to leveraging new AI and analytics technology to detect personal information is cost savings. The manual process of personal information identification is not only slower, but it can also be incredibly expensive. AI can significantly reduce the number of documents legal professionals would need to look through, sometimes by millions of documents. This can translate into millions of dollars in review savings because this work is often performed by legal professionals who are billed at an hourly rate.Not only can AI utilization save money on a specific matter, but it can also be used to analyze an entire legal portfolio so that legal professionals have an accurate sense of where (and how much) personal information resides within an organization’s data. This knowledge can be invaluable when crafting burden arguments for upcoming matters, as well as to better understand the potential costs for new matters (and thus help attorneys make more strategic case decisions).Another key benefit of leveraging AI technology is the accuracy with which this technology can now pinpoint personal data. Not only is human review much less efficient, but it can also lead to mistakes and missed information. This increases the risk for healthcare and pharmaceutical organizations especially, who may face severe penalties for inadvertently producing PHI or PII (particularly if that information ends up in the hands of malevolent actors). Conducting quality control (QC) with the assistance of AI can greatly increase the accuracy of human review and ensure that organizations are not inadvertently producing individuals’ personal information. Best Practices for Utilizing AI and Analytics to Identify Personal DataPrepare in Advance: AI technology should not be an afterthought. Before you are faced with a massive document production on a tight deadline, make sure you understand how AI and analytics tools work and how they can be leveraged for personal data identification. Have technology providers perform proof of concept (POC) analyses with the tools on your data and demonstrate exactly how the tools work. Performing POCs on your data is critical, as every provider’s technology demos well on generic data sets. Once you have settled on the tools you want to use within your organization, ensure your team is trained well and is ready to hit the ground running. This will also help ensure that the technology you choose fits with your internal systems and platforms.Take a Global Team Approach: Prior to leveraging AI and analytics, spend some time working with the right people to define what PII and PHI you have an obligation to identify, redact, or anonymize. Not all personal information will need to be located or redacted on every matter or in every jurisdiction, but defining that scope early will help you leverage the technology for the best use cases.Practice Information Governance: Make sure your organization is maintaining proper control of networks, keeping asset lists up to date, and tracking who the business and technical leads are for each type of asset. Also, make sure that document retention policies are enforced and that your organization is maintaining controls around unstructured data. In short, becoming a captain of your content and running a tight ship will make the entire process of identifying personal information much more efficient.Think Outside the Box: AI and analytics tools are incredibly versatile and can be useful in a myriad of different scenarios that require protecting personal information from disclosure. From data breach remediation to compliance matters, there is no shortage of circumstances that could benefit from the efficiency and accuracy that AI can provide. When analyzing a new AI tool, bring security, IT, and legal groups to the table so they can see the benefits and possibilities for their own teams. Also, investigate your legal spend and have other teams do the same. This will give you a sense of how much money you are currently spending on identifying personal information and what areas can benefit from AI efficiency the most.If you’re interested in learning more about how to leverage AI and analytic technology within your organization or law firm, please see my previous articles on how to build a business case for AI and win over AI naysayers within your organization.To discuss this topic more or to learn how we can help you make an apples-to-apples comparison, feel free to reach out to me at RHellewell@lighthouseglobal.com.data-privacy; ai-and-analyticsai-and-analytics, microsoft-365analytics; data-privacy; ai-big-data; bloglighthouse
Data Privacy
AI and Analytics
Blog

AI and Analytics: Reinventing the Privilege-Review Model
Identifying attorney-client privilege is one of the most costly and time-consuming processes in eDiscovery. Since the dawn of the workplace email, responding to discovery requests has had legal teams spending countless hours painstakingly searching through millions of documents to pinpoint attorney-client and other privileged information in order to protect it from production to opposing parties. As technology has improved, legal professionals have gained more tools to help in this process, but inevitably, it still often entails costly human review of massive amounts of documents.What if there was a better way? Recently, I had the opportunity to gather a panel of eDiscovery experts to discuss how advances in AI and analytics technology now allow attorneys to identify privilege more efficiently and accurately than previously possible. Below, I have summarized our discussion and outlined how legal teams can leverage advanced AI technology to reinvent the model for detecting attorney-client privilege.Current Methods of Privilege Identification Result in Over IdentificationCurrently, the search for privileged information includes a hodgepodge of different technology and workflows. Unfortunately, none of them are a magic bullet and all have their own drawbacks. Some of these methods include:Privilege Search Terms: The foundational block of most privilege reviews involves using common privilege search terms (“legal,” “attorney,” etc.) and known attorney names to identify documents that may be privileged, and then having a review team painstakingly re-review those documents to see if they do, in fact, contain privileged information.‍Complex Queries or Scripts: This method builds on the search term method by weighting the potential privilege document population into ‘tiers’ for prioritized privilege review. It sometimes uses search term frequency to weigh the perceived risk that a document is privileged.‍Technology Assisted Review (TAR): The latest iteration of privilege identification methodologies involves using the TAR process to try to further rank potential privilege populations for prioritized review, allowing legal teams to cut off review once the statistical likelihood of a document containing privilege information reaches a certain percentage.Even applied together, all these methodologies are only just slightly more accurate than a basic privilege search term application. TAR, for example, may flag 1 out of every 4 documents as privilege, instead of the 1 out of every 5 typically identified by common privilege search term screens. This result means that review teams are still forced to re-review massive amounts of documents for privilege.The current methods tend to over-identify privilege for two very important reasons: (1) they rely on a “bag of words” approach to privilege classification, which removes all context from the communication; (2) they cannot leverage non-text document features, like metadata, to evaluate patterns within the documents that often provide key contextual insights indicating a privileged communication.How Can Advances in AI Technology Improve Privilege Identification MethodsAdvances in AI technology over the last two years can now make privilege classification more effective in a few different ways:Leveraging Past Work Product: Newer technology can pull in and analyze the privilege coding that was applied on previous reviews, without disrupting the current review process. This helps reduce the amount of attorney review needed from the start, as the analytics technology can use this past work product rather than training a model from scratch based on review work in the current matter. Often companies have tens or even hundreds of thousands of prior privilege calls sitting in inactive or archived databases that can be leveraged to train a privilege model. This approach additionally allows legal teams to immediately eliminate documents that were identified as privileged in previous reviews.Analyzing More Than Text: Newer technology is also more effective because it now can analyze more than just the simple text of a document. It can also analyze patterns in metadata and other properties of documents, like participants, participant accounts, and domain names. For example, documents with a large number of participants are much less likely to contain information protected by attorney-client privilege, and newer technology can immediately de-prioritize these documents as needing privilege review.Taking Context into Account: Newer technology also has the ability to perform a more complicated analysis of text through algorithms that can better assess the context of a document. For example, Natural Language Processing (NLP) can much more effectively understand context within documents than methods that focus more on simple term frequency. Analyzing for context is critical in identifying privilege, particularly when an attorney may just be generally discussing business issues vs. when an attorney is specifically providing legal advice.Benefits of Leveraging Advances in AI and Analytics in Privilege ReviewsLeveraging the advances in AI outlined above to identify privilege means that legal teams will have more confidence in the accuracy of their privilege screening and review process. This technology also makes it much easier to assemble privilege logs and apply privilege redactions, not only to increase efficiency and accuracy, but also because of the ability to better analyze metadata and context. This in turn helps with privilege log document descriptions and justifications and ensuring consistency. But, by far the biggest gain, is the ability to significantly reduce costly and time-intensive manual review and re-review required by legal teams using older search terms and TAR methodologies.ConclusionLeveraging advances in AI and analytics technology enables review teams to identify privileged information more accurately and efficiently. This in turn allows for a more consistent work product, more efficient reviews, and ultimately, lower eDiscovery costs.If you’re interested in learning more about AI and analytics advancements, check out my other articles on how this technology can also help detect personal information within large datasets, as well as how to build a business case for AI and win over AI naysayers within your organization.To discuss this topic more or to learn how we can help you make an apples-to-apples comparison, feel free to reach out to me at RHellewell@lighthouseglobal.com.ai-and-analytics; chat-and-collaboration-data; ediscovery-reviewprivilege, analytics, ai-big-data, blog, ai-and-analytics, chat-and-collaboration-data, ediscovery-review,privilege; analytics; ai-big-data; bloglighthouse
AI and Analytics
Chat and Collaboration Data
eDiscovery and Review
No items found. Please try different search parameters.