What Attorneys Should Know About Advanced AI in eDiscovery: A Brief Discussion
October 12, 2021
By:
What does Artificial Intelligence (AI) mean to you? In the non-legal space, AI has taken a prominent role, influencing almost every facet of our day-to-day life – from how we socialize, to our medical care, to how we eat, to what we wear, and even how we choose our partners.

In the eDiscovery space, AI has played a much more discreet but nonetheless important role. Its limited adoption so far is due, in part, to the fact that the legal industry tends to be much more risk averse than other industries. The innate trust we have placed in more advanced forms of AI technology in the non-legal world to help guide our decision making has not carried over to eDiscovery – partly because attorneys often feel that they don’t have the requisite technological expertise to explain the results to opposing counsel or judges. The result: most attorneys performing eDiscovery tasks are either not using AI technology at all or are using AI technology that is generations older than the technology currently being used in other industries. All this despite the fact that attorneys facing discovery requests today must regularly analyze mountains of complicated data under tight deadlines.
One of the most prominent roles AI currently plays in eDiscovery is within technology assisted review (TAR). TAR uses “supervised” machine learning algorithms to classify documents for responsiveness based on human input. This classification allows attorneys to prioritize the most important documents for human review and, often, reduce the number of documents that need to be reviewed by humans. TAR has proven to be especially helpful in HSR Second Requests and other matters with demanding deadlines. However, the simple machine learning technology behind TAR is already decades old and has not been updated, even as AI technology has significantly advanced. This older AI technology is quickly becoming incapable of handing modern datasets, which are infinitely more voluminous and complicated than they were even five years ago.
Because the legal industry is slower to adopt more advanced AI technology, many attorneys have a muddled view of what advanced AI technology exists, how it works, and how that technology can assist attorneys in eDiscovery today. That confusion becomes a significant detriment to modern attorneys, who must start being more comfortable with adopting and utilizing the more advanced AI tools available today if they stand a chance overcoming the increasingly complicated data challenges in eDiscovery. This confusion behind AI can also lead to a vicious cycle that further slows down technology adoption in the legal space: attorneys who lack confidence in their ability to understand available AI technology subsequently resist adoption of that technology; that lack of adoption then puts them even further behind the technology learning curve as technology continues to evolve.
This is where legal technology companies with dedicated technology services can help. A good legal technology company will have staff on hand whose entire job it is to evaluate new technology and test its application and accuracy within modern datasets. Thus, an attorney who has no interest in becoming a technology expert just needs to be proficient enough to know the type of tools that might fit their needs – the right technology vendor can do the rest. Technology experts can also step in to help provide detailed explanations of how the technology works to stakeholders, as well as verify the outcome to skeptical opposing counsel and judges. Moreover, a good technology provider can also supply expert resources to perform much of the day-to-day utilization of the tool. In essence, a good legal technology vendor can become a trusted part of any attorney team – allowing attorneys to remain focused on the substantive legal issues they are facing.
With that in mind, it’s important to “demystify” some common AI concepts used within the eDiscovery space and explain the benefits more advanced forms of AI technology can provide within eDiscovery. Once comfortable with the information provided here, readers can take a deeper dive into the advantages of leveraging advanced AI within TAR workflows in our full white paper – “TAR + Advanced AI: The Future is Now.” Armed with this information, attorneys can begin a more thoughtful conversation with stakeholders and legal technology companies regarding how to move forward with more advanced AI technology within their own practice.
Demystifying AI Jargon in eDiscovery
At its most basic, AI refers to the science of making intelligent machines – ones that can perform tasks traditionally performed by human beings. Therefore, AI is a broad field that encompasses many subfields and branches. The most relevant to eDiscovery are machine learning, deep learning, and natural language processing (NLP). As noted above, the technology behind legacy TAR workflows is supervised machine learning. Supervised machine learning uses human input to mimic the way humans learn through algorithms that are trained to make classifications and predictions. In contrast, deep learning eliminates some of that human training by automating the feature extraction process, which enables it to tackle larger datasets. NLP is a separate branch of machine learning that can understand text in context (in effect, it can better understand language the way humans understand it).
The difference between the AI technology in legacy TAR workflows and more advanced AI tools lies in the fact that advanced AI tools use a combination of AI subsets and branches (machine learning, deep learning, and NLP) rather than just the supervised machine learning used in TAR.
Understanding the Benefits of Advanced AI
This combination of AI subsets and branches used in advanced AI tools provides additional capabilities that are increasingly necessary to tackle modern datasets. These tools not only utilize the statistical prediction that supervised machine learning produces (which enables traditional TAR workflows), but also include the language and contextual understanding that deep learning and NLP provide. Deep learning and NLP technology also enable more advanced tools to look at all angles of a document (including metadata, data source, recipients, etc.) when making a prediction, rather than relying solely on text. Taking all context into consideration is increasingly important, especially when making privilege predictions that lead to expensive attorney review if a document is flagged for privilege. For example, with traditional TAR, the word “judge” in the phrases, “I don’t think the judge will like this!” on an email thread between two attorneys and, “Don’t judge me!” on a chat thread with 60 people regarding a fantasy football league will be classified the same way – because statistically, there is not much difference between how the word “judge” is placed within both sentences. However, newer tools that combine supervised machine learning with deep learning and NLP can learn the context of when the word “judge” is used as a noun (i.e., an adjudicator in a court of law) within an email thread with a small number of recipients versus when the word is being used as a verb on an informal chat thread with many recipients. The context of the data source and how words are used matters, and an advanced AI tool that leverages a combination of technologies can better understand that context.
Using Advanced AI with TAR
One common misconception regarding using newer, more advanced AI tools is that old workflows and models must go out the window. This is simply not true. While there may be some changes to review workflows due to the added efficiency generated by advanced AI tools (the ability to conduct privilege analysis simultaneously with responsive analysis, for example), attorneys can still use the traditional TAR 1.0 and TAR 2.0 workflows they are familiar with in combination with more advanced AI tools. Attorneys can still direct subject matter experts or reviewers to code documents, and the AI tool will learn from those decisions and predictive responsiveness, privilege, etc.
The difference will be in the results. A more advanced AI tool’s predictions regarding privilege and responsiveness will be more accurate due to its ability to take nuance and context into consideration –leading to lower review costs and more accurate productions.
Conclusion
Many attorneys are still hesitant to move away from the older, AI eDiscovery tools they have used for the last decade. But today’s larger, more complicated datasets require more advanced AI tools. Attorneys who fear broadening their technology toolbox to include more advanced AI may find themselves struggling to stay within eDiscovery budgets, spending more time on finding and less time strategizing – and possibly even falling behind on their discovery obligations.
But this fear and hesitancy can be overcome with education, transparency, and support from legal technology companies. Attorneys should look for the right technology partner who not only offers access to more advanced AI tools, but also provides implementation support and expert advisory services to help explain the technology and results to other stakeholders, opposing counsel, and judges.
To learn more about the advantages of leveraging advanced AI within TAR workflows, download our white paper, “TAR + Advanced AI: The Future is Now.” And to discuss this topic more, feel free to connect with me at smoran@lighthouseglobal.com.
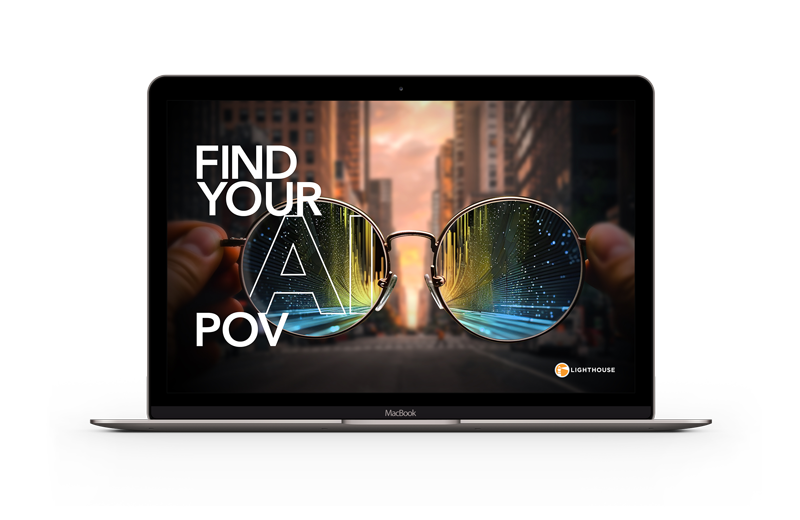