How AI Enhances Document Review
September 30, 2024
By:
Summary: AI can support a variety of tasks in document review, improving accuracy, consistency, and efficiency. Learn how to use it and what's in store for the future.
AI is rapidly disrupting how legal teams think about eDiscovery, and no aspect is more apt for change than document review.
But how exactly can AI change the way that legal teams work today? And what are the benefits?
To answer those questions, first we’ll break down the two main types of AI and how they work. Then we’ll explore what they can already do for you today—and game-changing features in development for the future of AI and document review.

The role of AI in eDiscovery and review
The latest boom in AI is driven by advancements in large language models (LLMs). This is the technology behind ChatGPT and other AI tools that analyze and produce language in an extremely sophisticated way, which mimic human communication.
LLMs enable two types of AI that play an increasingly important role in document review.
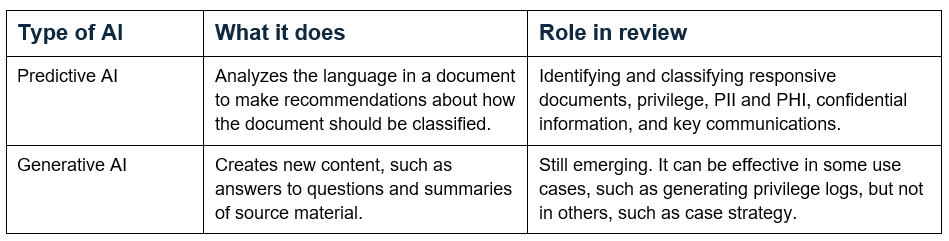
How predictive coding works
There are two main steps to using predictive AI to help classify documents.
First you train the predictive AI model
The legal team creates a sample set of documents to train the AI model. For things like responsiveness and relevant issues which are specific to each matter, this means reviewing and coding a subset of documents for the current dataset. For something like PII, which is defined the same way across matters, teams can leverage AI databanks and work product from prior matters.
The sample documents and support data are fed to the AI model to “teach” it what to look for when analyzing new documents.
Then the AI model applies what it’s learned
The AI model analyses the rest of the dataset and gauges how likely each document fits the classification at hand. This likelihood is expressed as a percentage. For example, AI may determine a document is 89% likely to be privileged, based on the content of the document and how it compares to the sample documents. The model will continue to fine tune as decisions are made during the document review.
Attorneys use those likelihood percentages to make strategic choices about how to proceed with manual review. To best take advantage of the AI models, using the most up-to-date technology with LLMs in strategic workflows should be top of mind. By considering alternate resource levels based on categorization combined with bypassing layers of review that have become redundant by the current technology, you can achieve higher quality outcomes in less time—avoiding significant cost.
How Generative AI works in eDiscovery
Broadly speaking, generative AI produces (or generates) content in response to a prompt. Unlike predictive AI, instead of training the model on additional data, the focus is on crafting the right prompt to obtain the desired output.
Creating an effective prompt is more complex than it may seem at first glance. Gen AI uses math to generate language, and a correctly formed prompt will provide the parameters and directions the technology needs to provide an accurate answer.
Gen AI responds with words and sentences that are statistically likely to satisfy the prompt. That is, gen AI doesn’t compose a response by weighing the information at hand. Instead, it analyzes the language it has been given and—based on the millions of examples it was initially trained on—builds an appropriate response one word at a time.
Given the need for precise language in gen AI prompts, it’s important to use it in ways that avoid open-ended or ambiguous questions, particularly for eDiscovery. Generating privilege logs is an example where the prompts and efficacy of AI are straightforward. Once you ask gen AI to create a privilege log line, you can ask how well it explained why the doc is privileged.
Why use case matters with gen AI
The more open-ended the use case for gen AI, the more vulnerable it is to responding in ways that satisfy the prompt but stray from reality. This is why it’s risky to use gen AI for case building and strategy without the right prompting and validation techniques. And while it can be used for document classification, it is fundamentally not focused on precision the way that predictive AI is.
In controlled and targeted use cases, gen AI can be quite helpful. Again, this is why privilege logs are a great example. A gen AI solution can generate defensible privilege log and redaction log descriptions that meet the requirements provided in the prompt.
Human oversight is the key factor. Crafting prompts for gen AI in eDiscovery is a complex and often iterative process, best performed by experts who know how to guide AI models to generate accurate outcomes. And a human should always play the role of reviewing and approving AI-generated content, rather than taking it at face value.
Key benefits of AI integration
Predictive and generative AI models support a marked evolution of a process which has remained relatively stagnant for many years. Strategic integration of AI models can empower legal teams with early insight, scale efforts for large matters, manage complex datasets and short deadlines, and get the most out of their eDiscovery budget.
Enhancing accuracy and consistency
Even at their best, traditional review methods are prone to errors. This can range from inadvertently producing protected information, missing key information critical to a merits assessment, or necessitating additional cycles of quality control to correct issues.
Modern AI has proven to be more accurate, particularly for today’s large datasets. When set up and implemented appropriately, gone are the days of reviewing the masses of false positive privilege documents at the expense of targeting those that may be more easily missed by humans reviewing at a fast pace or basic search terms.
For example, an AI privilege classifier helped one of our clients avoid reviewing more than 100K documents that had hit on privilege search terms but weren’t found to be privileged.
This accuracy can also be applied matter over matter—leveraging prior work product for greater accuracy and consistency on future matters—particularly where sensitive data and/or sanctions are at issue.
Increasing efficiency in document review
Modern datasets are so large and complex that a new breed of technology solutions are necessary to boost efficiency.
Modern AI helps increase efficiency in multiple ways:
- Reducing hours required by human reviewers typically by 25-40%
- Combining processes which were formerly sequential into concurrent actions
- Minimizing eDiscovery spend matter-over-matter with an impactful ROI legal departments and law firms can control
Best of all – modern AI may help you gain a tactical advantage earlier in the process
It’s easy to see that AI solutions for our industry will continue to grow in adoption and utility over the next few years. Changes are happening rapidly, and it can be hard to keep up. To learn more about the aspects and opportunities of modern AI, check out our AI at Lighthouse.
Or get in touch with us. We’re always ready to talk about what’s possible.
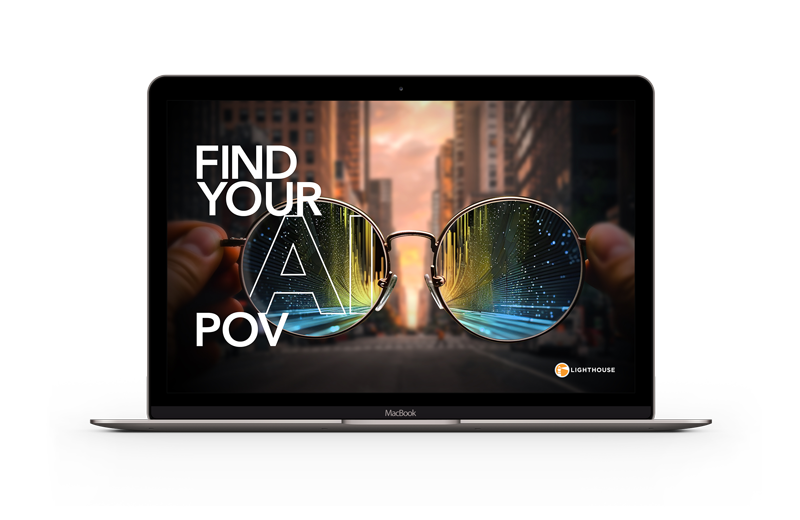