How AI Can Streamline Second Requests
December 5, 2024
By:
Summary: Second Requests, while challenging, are driving the adoption of cutting-edge AI technologies in legal document review. Discover how you can successfully utilize large language models (LLMs) and generative AI to improve your process and accuracy on these matters.
Hart-Scott-Rodino Second Requests are notorious for being pressure cookers. With aggressive deadlines, massive data volumes, and high-stake M&A deals on the line, these antitrust investigations by the DOJ and FTC put intense stress on in-house counsel and the law firms supporting them. It’s hard to imagine any attorney thinking about the process in a positive light.
However, there is an often-overlooked benefit of Second Requests: The process tends to drive early adoption of technology that makes document review more efficient and accurate. The high data volumes and short turnaround times push legal teams towards tech that can help them meet substantial compliance—without the fear of “discovery on discovery” that often comes with civil litigation.
Learning how to leverage technology like AI on one Second Request not only helps you streamline future Second Requests, but it can also give you an overall competitive edge as you transfer your AI experience to other types of matters.
Below, we’ve put together a FAQ for curious attorneys who want to understand how innovative legal teams are using AI in Second Requests to gain a competitive advantage.
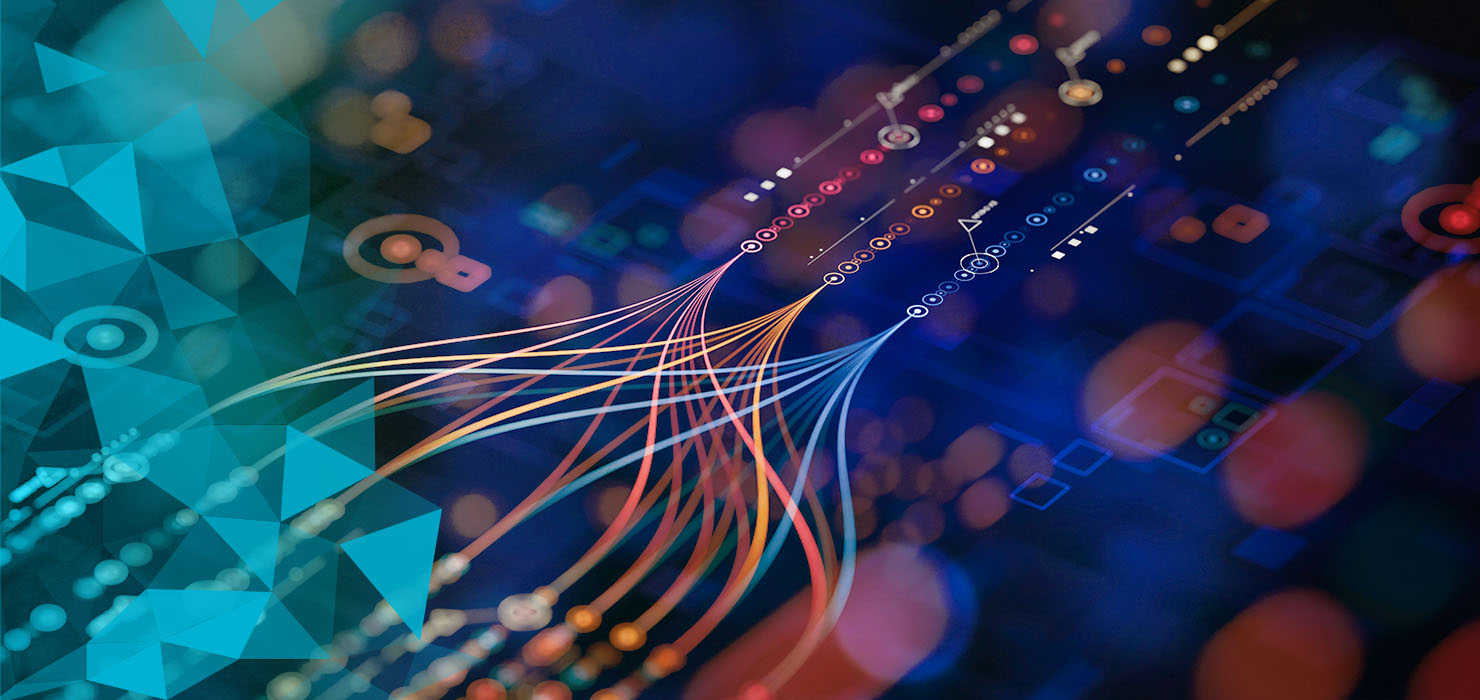
What types of AI technology are attorneys using in Second Requests?
Attorneys are increasingly using predictive and generative AI capabilities that utilize LLMs in Second Requests. AI-backed by LLMs is proving to be incredibly adept at handling the high data volumes and tight deadlines inherent to most modern Second Requests.
What are LLMs? And how are they different from TAR?
LLMs are advanced AI systems that analyze language as it is used in real-world contexts. These models mark a significant evolution from the traditional machine learning tools typically used in TAR workflows.
Classical machine learning tools employ techniques like word frequency analysis to interpret data. In contrast, LLMs interpret words as interconnected data points, whose meanings shift based on their usage. For instance, LLMs understand that the word "train" has different meaning in the sentence "I have a train to catch" versus "I need to train for the marathon."
This nuanced understanding of language was popularized by generative AI platforms like ChatGPT—but LLMs are foundational to both predictive and generative AI applications.
What’s the difference between predictive AI and generative AI?
Predictive AI focuses on forecasting truths about the present or future by analyzing extensive data sets—like predicting weather patterns or a user’s streaming preferences. These predictions are probability-based, emphasizing output precision.
Generative AI creates new content based on existing examples within the data set it was trained on. For instance, when ChatGPT responds to a query, it is not merely recalling facts but generating new text based on vast internet-sourced data, without prioritizing precision. This is the reason that generative AI output must be closely examined and corrected for incorrect responses; it focuses on creating over accuracy of results.
Can legal teams use LLMs to enhance TAR workflows in Second Requests?
Yes. Integrating LLMs with predictive TAR workflows allows legal teams to refine responsiveness and privilege models more swiftly and with fewer iterations. This integration enhances precision and recall, offering eDiscovery practitioners an unprecedented level of efficiency and scalability in traditionally cumbersome TAR workflows. The exacting timelines and voluminous data inherent in Second Requests make this advanced TAR approach particularly desirable.
Are legal teams using generative AI in Second Requests yet?
Yes. Innovative legal teams are applying generative AI to optimally sized document populations, enhancing processes such as privilege logging and further easing the load on antitrust counsel. So far, we have seen use cases such as QC of privilege assessments and summarization of documents. This application of AI not only mitigates risks but also reduces the overall burden on legal teams during critical antitrust reviews.
Generative AI is also increasingly being used to improve seed set identification during the initial training of TAR models. This application allows for a more robust set of training documents, enhancing accuracy and reducing the number of necessary training iterations. By ensuring higher model performance, generative AI decreases the risks and costs associated with processing non-relevant documents in later stages.
If I want to start integrating more advanced AI during Second Requests, where’s the best place to start?
The key to integrating advanced AI into Second Requests is to lay the groundwork now. Look for trusted eDiscovery providers who have the technology and expertise available to help your team adeptly leverage LLM-backed AI tools in Second Requests. You should also invest in technology training for yourself and other attorneys and staff that support Second Requests.
Conclusion
As legal teams continue to confront the challenges posed by Second Requests, the role of AI—especially LLM-based technologies—will play an increasingly pivotal role. Attorneys who learn how to leverage that technology now will reap competitive advantages down the line.
Learn more about how Lighthouse is helping legal teams leverage technology in Second Requests on our antitrust practice page.
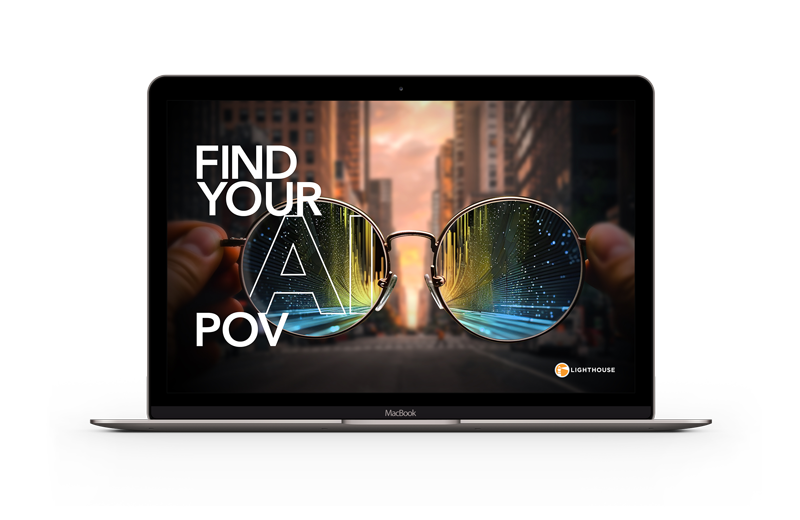